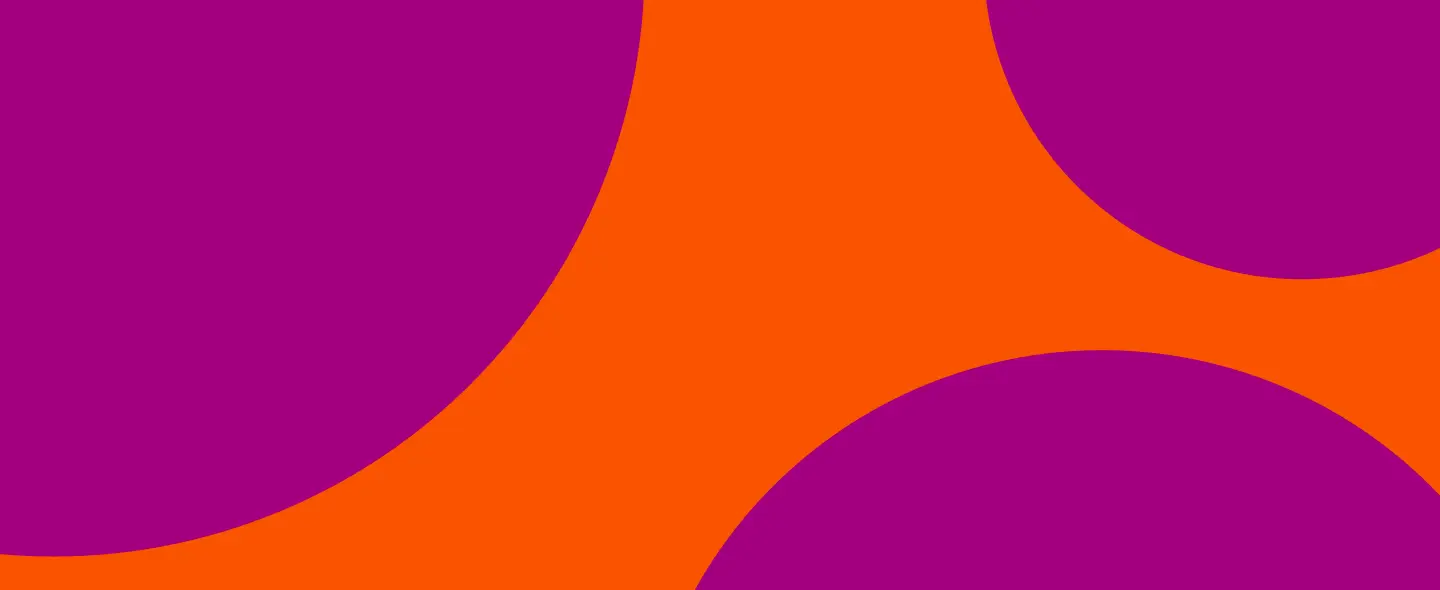
The Future of Biometric Security: Exploring Iris and Facial Recognition with Marios Savvides - Part 1
Share
Podcast
About This Episode
In today's episode, we're thrilled to dive deep into the fascinating world of biometric security with our special guest, Professor Marios Savvides from Carnegie Mellon University. Marios is not only a leading expert in artificial intelligence and biometric technology but also the founder and director of the Biometric Center, and he was named Inventor of the Year in 2022 by the Pittsburgh Intellectual Property Association. We'll explore a range of intriguing topics, including the exceptional robustness of iris recognition technology, advancements in non-intrusive biometric systems, and the critical role of human-computer interaction in security.
Marios will share insights on overcoming challenges in iris and facial recognition, tackling biases in AI, and the ethical implications of AI decision-making, especially in autonomous vehicles. We'll also touch on pressing privacy and security concerns, such as the impact of facial recognition in public spaces and the emerging threat of deep fakes.
Podcast
Popular Episodes
Podcast
The Future of Biometric Security: Exploring Iris and Facial Recognition with Marios Savvides - Part 1
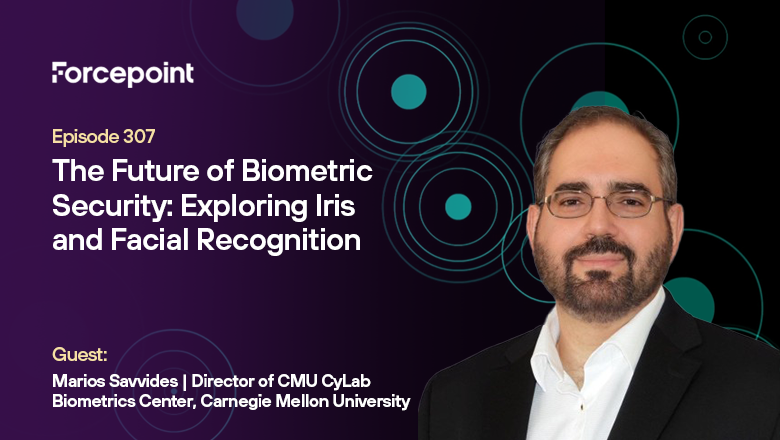
Rachael Lyon:
Welcome to To The Point cybersecurity podcast. Each week, join Vince Spina and Rachel Lyon to explore the latest in global cybersecurity news, trending topics, and cyber industry initiatives impacting businesses, governments, and our way of life. Now let's get to the point. Hello, everyone. Welcome to this week's episode of To the Point podcast. I'm Rachel Lyon here with my cohost, Vince Spina. Vince, hello. Hello.
Vince Spina:
Rach. How are you doing?
Rachael Lyon:
I'm doing well. How are you? I I know this is going to go live way after Halloween, but, did you do anything fun for Halloween?
Vince Spina:
No. I got to tell you, I'm disappointed. So, for the listeners, I moved from the Bay Area, now I'm in Arizona. In the Bay Area, we would get and this is without exaggeration 12, 13, 1400 trick or treaters in about a 3 to 4 hour span. I mean, they just kept coming in droves and droves. Here, I got exactly no trick or treaters. And, we purchased quite a bit of candy, thinking, you know, it wouldn't be as popular where we're at now as it was in our in our, last residence, but literally 0. And my wife's like, you better get rid
Vince Spina:
of that candy. Like, we didn't have 1, not 1,
Vince Spina:
and, so it was a little bit disappointing, to be honest.
Rachael Lyon:
Well, were the the lights off at your house, or was it an inviting okay.
Vince Spina:
Yeah. No.
Vince Spina:
It's a it's a newer neighborhood, and, there there's space between houses. So I think in the, you know, the 6 year old efficiency model, they're like, yeah. It's not worth going down that cul de sac where there's 2 homes, you know, a good distance apart from each other. We're we're we're gonna stay where it's a little more dense in houses. That makes sense. It's critical mass. Efficiency thing.
Rachael Lyon:
Yeah. Exactly. Exactly.
Vince Spina:
They did a motion and time study. I'm trying to impress Mario's here, but, I think, you know, I think I think these 6 year olds are industrial engineers. They were doing some motion and time and said, yeah. We're gonna stay away from that street and and just keep moving on.
Rachael Lyon:
That's right. Time is candy. Time is candy.
Vince Spina:
Time is candy.
Rachael Lyon:
Absolutely. Oh
Vince Spina:
my god. That might stick. That might that one might stick.
Rachael Lyon:
Well, I'm so excited for today's guest. Everyone, please welcome, professor Marios Savides. He holds the Bossa Nova Robotics Professorship of AI at Carnegie Mellon University. He's also the founder and director of the Biometric Center and a full tenured professor in electrical and computer engineering. And if that's not enough, he also, in 2022, was named inventor of the year by the Pittsburgh Intellectual Property Association for his contributions to the advancement of AI and biometric technologies. Wow. Marios, welcome.
Marios Savvides:
Thank you, Rachel. Thank you, Vince. It's an honor to be on your podcast, and I just, thank you for the opportunity. It's it's my pleasure to be here. Thank you.
Rachael Lyon:
This is gonna be a fun conversation. Vince, do you want to kick off
Vince Spina:
the first question? Yeah. I'll kick it off, and it is gonna be fun. And I'll tell you, you know, Morris, we try to keep it to about 45 minutes. This one could go for 45 hours,
Marios Savvides:
I think. I mean, the the kinda
Vince Spina:
the topics that we wanna kinda dig in. This is real time today. People care about it both in their personal lives, their professional lives. So, if you don't mind, let's start with facial detection. And Rachel and I, our company and a lot of our listeners are in the world of, cybersecurity. So, you know, first question maybe for you is from a facial detection perspective, what role do you think that technology plays in enhancing, our listeners' cybersecurity solutions? And particularly, you know, some of the technologies we care about are things like authentication, access control, you know, etcetera. So what's your thoughts on that?
[03:54] Facial Recognition's Weakest Link: Face Detection
Marios Savvides:
It's a great question, Vince. So, you know, in in facial recognition, if we if we think about the whole workflow, the first thing an an AI system does is detect faces. So it has to do facial detection. And so when you think of it from a security point of view, that is the weakest link. If I can't detect a face, I'm not even trying to authenticate or recognize someone. So many times, you know, adversarial attacks will try to actually, you know, fool detection systems so that someone goes completely undetected. And it's been a big problem. And pre COVID, you know, most of the commercial technologies would not even detect faces that were just masks.
Marios Savvides:
Somebody was wearing a mask, even a COVID mask, it would not find a face. You know, we worked on that problem almost 10 years ago, and we saw that way before COVID, that if you think about faces, you gotta find a face. It could be partial face. It could be a face behind a face. Right. Right. Somebody's just wearing a hood and wearing a cap, baseball cap. It's really lower down.
Marios Savvides:
So detecting faces is is has to be extremely robust because the moment you you you you fool that, you now are you're you're invisible to the system.
Vince Spina:
Morris, I'm I'm gonna keep going around. I just wanna pull that thread because that's interesting. Like, can you define what makes a face? Like, where does it start? Where does it stop? And I'm thinking even just like on my iPhone in that, my iPhone is letting me in now Right. Even where I wear glasses or sometimes sunglasses or things like that. And I'm like, well, how did it learn that the face is still there, but, you know, I'm covering up the eyes or something? So what what is a face today, I guess, is the question like?
Marios Savvides:
You know, that's a great question. Because a face in the old days was you had to had 2 eyes, a nose, and a mouth. And if some part of that wasn't there, you couldn't find a face. I mean, now a face is, like I said, anything. Right? Your eyes could be blocked because you're wearing sunglasses. Does that mean there's no face? You have no eyes. Right? The system doesn't find eyes. It still has to find a face.
Marios Savvides:
So the mouth is there. The nose is there. Even if there's just one eye, that's still part of a face. You still have to authenticate or you have to recognize. So, the good thing is AI systems have gotten stronger. We've built our technologies to make it, you know, we can find a face even if you literally just this part of the eye is just visible. And it's important because you and I, the gap has been can we develop AI systems that can detect a face we are as good as humans, right? Because even if you see somebody peeking through and you just see one eye. Oh, there's someone's over there.
Marios Savvides:
I see an eye. Well, I see an ear. I see part of a face. Most of the AI before, I would say you know 5 years ago was not there. Now, it's there. We can find a face as good as people do. So, and it's very important. Again again, it's it doesn't mean you will recognize it always.
Marios Savvides:
Right? If I just see one eye or I just see part of an ear. Doesn't mean I can recognize who it is. Although, there is technology that you can use to do some ear recognition or we've done some periocular work. But But at least you know there's a face and you start looking, okay, well, I can track. Well, okay. Now, I see a partial view. When am I gonna see more of you and then I can recognize it. Right? So when do I queue in the recognizer, let me extract the face.
Marios Savvides:
Or, you know what? I'm not gonna trust the recognition because I only see 5% of a face. So I'm not gonna trust what it tells me because I just there's just no information. So knowing the knowing how much of the face is there, knowing the face is there, knowing if it's occluded, if it's masked, if it's someone's painted, if, you know, knowing those extra attributes are important key features to go into the recognizer so that it gives a more informed decision. Just like you and I looking at someone or I see an eye. You know what? That looks like Marius. I'm not sure. And the more you see that, oh, that is Marius. We have to make the AI systems mimic that human thought process.
Marios Savvides:
Oh, I have more information. Oh, now I'm sure that is how do I accumulate that data? So AI systems are getting smarter so that we're using that extra information to come up with something equivalent to how we would actually organize someone.
Rachael Lyon:
Yeah. It's amazing how far it's come. You know? And one of the things that you kinda keep hearing and, you know, kind of in in discussion too about, you know, hold the facial recognition is what are privacy and security concerns, right, with the use of facial detection technology, particularly in public or semipublic spaces. I I think of the airport now. Instead of, I think checking your boarding ticket or whatever, they verify you with, your face. You look into the little, you know, recognizer, and then it either recognizes you or you or it doesn't. But I just thought that was fascinating. When did that get set up, and how did it get my my face? So I'd be interested in your perspective here on that that conversation.
Marios Savvides:
Yeah. So, you know, airports are a different scenario. Right? I mean, they're, it's a public space, it's, it's more for, you know, security. You have, you know, you're going through a system that uses facial recognition, right?
Rachael Lyon:
Right.
Marios Savvides:
I think to me, what is more interesting is what happens in outside, you know, public spaces
Rachael Lyon:
Right.
[09:13] Illinois' BIPA Ensures Biometric Privacy, allows Lawsuits
Marios Savvides:
When there is in public spaces, there's no notion of, privacy. Right? At least expected. But if you are somewhere else under a commercial, how is your information being used? Right? Do you is there consent? Right? And when I think about those things, you know, lately, I've been seeing a lot of, things going on, particularly in the state of Illinois with if you think about the BIPA act, the, you know, the biometrics information privacy act. Right? Illinois is one of the states that really has gone very far to make sure to protect the the biometric privacy of individuals. And, you know, what information is being collected, stored, and it it has given the right to users to sue companies that, you know, are collecting biometrics without their consent. And and it's, it's very interesting. And I and I think that's also a place where the law may have gone the other direction. I think it has become vague on hope where, you know, a lot of users are trying to sue anything that they may be using biometric or maybe using a face, even though they're not really doing facial recognition.
Marios Savvides:
They're doing something completely different. So, you know, with everything, there's a saying in Greek, which means, do everything with moderation. Right? Allah is there to protect and it's make sure that, you know, nothing is abused. But at the same side, it should not be abused by the users as well. It's very interesting how I see all these things evolve, and, yeah, it it's it's interesting. Morris,
Rachael Lyon:
I'm sorry.
Vince Spina:
I think I probably won't ask you, like, to me, there's, boy, there's a whole conversation around, the ethical considerations when it comes to, you you know, this this type of technology. Probably stay away from that, but what about on the bias side when it comes to things like gender, ethnicity, you know, things like that. Does how does that all play into facial, detection? Is there
Marios Savvides:
Vince, great question. Right? I mean, I'm and and that's where, you know, unfortunately, biometric technology has taken a lot of heat. Right? There was a study with MIT and Microsoft. I think it was called the gender shades project. Right? Where they basically examined 3 facial recognition systems from IBM, Microsoft, and Face plus plus And what it showed was that it recognized males better than females. And the the performance gap was anywhere from 8% to 20% difference. Right? They even did analysis on on just the the folks, you know, the lighter lighter skin subjects versus dark skinned. And it showed that the performance gap between those systems range between 11% to almost 19%.
Marios Savvides:
Wow. So and and those are just those systems. Right? I'm not saying now. And and then even when we train systems, we make sure things are, there's that the bias is eliminated. But the way you try to eliminate bias is that 2 ways. You have to make sure that when you train an AI system or a facial training system, you have an equal distribution of your data. Meaning, if you, for any reason, do you have more subjects for one particular ethnic group than another, an AI algorithm will naturally tend to work better for what it's seen more of. Right? And it may not care.
Marios Savvides:
So if I have, you know, 10% error, it could be that, you know, there's a lot more error in that bias. So you have to make sure the accuracy is not just an overall accuracy, but rather it is an accuracy that I'm I'm striving to get 99% accuracy. And it has to be 99% on all ethnic groups and general groups. Right? So that when I'm quoting accuracy, I've specifically made sure my algorithm is not biased, right? So it was kind of a wake up call. It's like, okay, woah. Yeah. One number is not good enough. I have to make sure that my system, anyone's system is equally working well for all gender, age, ethnic groups, right? And I think it was a very good and this did a similar study as well and showed that.
Marios Savvides:
So it was a wake up call for researchers and commercial entities to pay attention to those things. And there was also that whole Google algorithm that wasn't, was detecting really strange kind of faces. Right? And that wasn't good. Now yeah. It it it it's important. And sometimes people will discover something as a, oh, look at this, and things are blown out of proportion. Reality is it does make an error and should minimize and look at and fix those errors. That's very important.
Marios Savvides:
So reducing bias is is is now an ethical responsibility of of AI scientists. Right? It has to be. And you know, when you talk about ethics, it it it's also interesting when you think about, you know, that's facial recognition. Think about now a car. Autonomous car. It's driving, it's driving in the middle of the road. It may not be able to stop, and maybe it has to make a turn. I'm just making this up.
Marios Savvides:
Sure. Okay. And and there's 2 people. Like, there's an elderly gentleman, you know, a grandfather and a young kid. Unfortunately, he will be
Vince Spina:
Carlos is about to go Sophie's choice on something. Oh, yeah.
[14:52] AI Decision-Making in Edge Cases is Crucial
Marios Savvides:
Yeah. You know, this is a few more things. We we systems. We have to think about edge cases. Right? And and it's very important that, you know, AI systems what do you do? What do you do? What does an AI system in all these edge cases? And someone's making a decision somewhere. Do you save the person in the car, or do you save the person not in the car? Because at the end of the day, you know, you may have to hit something, and that may kill the person in the car. Or, you know, whoever jumped in the middle of the road. Which is why you need a lot of good, not only AI, but sensors to be able to detect pedestrians and see if someone is on a trajectory to jump in front or do something.
Marios Savvides:
Right?
Rachael Lyon:
Yeah. It's it's a tough one.
Marios Savvides:
I mean,
Rachael Lyon:
I will say just, to sidebar, Mario, so when I lived in New York City, you know, driving through certain areas and, you know, the Bronx or whatever, it would be dark and people would be in between cars. You wouldn't see them, and they would just come out of nowhere. And if you're not driving really slow, you know, it's it's a problem. And, you know, and those are, you know, kinda real world challenges, right, with any kind of autonomous vehicle. You know, I think the sensors are critical, but how fast can they respond, you know, unless you're always going 10 miles an hour.
Marios Savvides:
Right. I'll tell you. I, I I live up north in here in Pittsburgh, and we get a lot of deer. So I installed a thermal camera. It's like a deer alert. But it's not just a deer alert. It also sees anything that's, you know, has a high heat signature. So in oh, and and, Rachel, like you said, I drive I go to New York sometimes, and at night or people will jump in, and you don't see them because they just know it's some sometimes you're in a neighborhood or area that's it's very dark.
Rachael Lyon:
Yes. But
Marios Savvides:
that system actually has helped me detect when there's a pedestrian where I wouldn't see, woah, there's somebody there. I can't see because maybe they're just, you know, wearing very dark clothes anyway, and it's not very well lit in that area. So this is where AI and good sensors can actually help. So if done right, I think they actually can assist because that's assisted me. Ordinarily, I wouldn't even have seen that there's a person about walking. Like, woah, where did this guy come from? Like, oh, I saw them in my thermal camera because it was this bright heat map of of of I don't know, of a a thermal object.
Rachael Lyon:
Wow. I didn't even know you could get such a thing for the car.
Marios Savvides:
We can. It's a night vision. The the cars have night vision. Some cars you can and you cannot you can retrofit.
Rachael Lyon:
Oh, wow. Okay. I like that. I need to I need to get on that. That's genius. So we can't have a conversation about facial detection, and recognition without bringing in the AI element, right, that everybody talks about. And, you know, with with AI kind of, you know, growing and evolving and and doing all the things, you know, there there have been conversations as well, right, on the ability to replicate a voice or replicate a face in AI. And so how can, you know, facial detection systems differentiate? How do how can they know what's real and and what's not real if if someone were trying to, you know, kind of, use my face to get into a certain system thinking that I have, you know, privileged access or something like that.
Marios Savvides:
That's a great point, Rachel. So I think you're you're you're touching upon 2 points. 1 is the real world authentication aspect. You know, can somebody do a, you know, a Tom Cruise Mission Impossible phase spoof, right, to get access to a system? And the way people do that, or at least, detect spoof attacks is well, there's 2 things. What's the how can someone attack? Someone will attack by maybe having a printout, you know, a picture of a person. Right? Mhmm. That looks exactly the same. But then you start looking at, okay, well, is this a picture? Meaning, is this a flat surface versus a 3 d face.
Marios Savvides:
Right? So, if you're using something like, you know, one of those Intel RealSense cameras or any camera that can detect depth, right? Or 3 d stereo. Then you can say, well, okay. I have a 3 d object. This is not somebody displaying an iPad with someone's face. This is something that actually is a 3 d. Then the question is, is somebody wearing a mask or not that looks at someone? And then the question is, can you detect the pulse? A mask won't have that pulse signature. So, basically cameras are sensitive enough that can detect something we can't see. So blood is flowing through our whole body and it's flowing through our head.
Marios Savvides:
And you can actually detect very weakly in in in the camera, the color the color fluctuates very minutely. And you can actually start extracting a heart rate just by looking at the camera and the person's face. And so, if you if you ploy some of those methods, then you can detect, well okay, is this somebody wearing a mask? Or is that somebody is that a real person with blood flowing through their head? So that's how you can sort of solve the presentation attacks to an actual physical access system. Now, I think another question you were alluding to is, well, in the digital world now we have deep fakes. Is this social media, is this viral video of whatever we're seeing, Is that that person or is that a deep fake of the person?
Rachael Lyon:
Right.
Marios Savvides:
Right? So, misinformation. Mhmm. And that gets tricky. Because you need, A, you need temporal information, right? Deepfakes are going so good that from just a single image, you can't tell it to deepfake or not. And one of the giveaways that most deepfakes can be detected is the eye movement. If you look at a, you know, a spoof and there's many of these, you know, people acted become, you know, Tom Cruise or whatnot. And they look amazingly realistic, right? And I can send you some videos I teach in class and I show them. Like students are like, wow, that's like looks like him.
Marios Savvides:
And even the voice sounds like him too. Yes, yes. But the eyes, if you look closely at the eyes, there's gaps. The eye movement is not natural. Where they're looking at is not natural. And sometimes it looks like they're looking at, you know, their eyes are not converging on real objects. That that yes. We can detect if we look at it.
Marios Savvides:
And those are some of the giveaways that you can tell if something's a deep fake or not. I mean there's many other things. There's many other AI that start looking at frequency information to figure out if that's a deep fake or not. But you know, the the eye blinking, the eye movement is something that we can also visually say, oh, there's something not exactly right here. So the consistency, basically, the temporal consistency is not there. You'll see something change. You'll see some facial features maybe appear, disappear, those kind of things.
Vince Spina:
Yeah. Morris, that's a great segue, I think. So talking a little bit about the eyes, you know, another technology that's, at the forefront, in this, you know, realm is the notion of virus caption excuse me, capture technology. You know, how does that compare in your opinion? It sounds like it's fairly favorable, but, relative to other biometric systems, in terms of things like, resistance to spoofing, fraud, you know, things like that. You know, it sounds like, you know, you're on the side of this is pretty favorable technology. Why don't you tell us a little bit about that?
[22:44] Iris: Unique, Stable, Lifelong Identifier like Fingerprints
Marios Savvides:
Oh, yeah. I love iris, and we we have a long history of iris. So iris is basically, you know, iris is the sphincter muscle between the pupil and the sclera, the white part of the eye, and it's the muscle that controls the pupil to dilate or contract. And that muscle, the iris pattern is actually unique to every individual. More importantly, it's thought not to change over a person's lifetime just like a fingerprint. So face ages, we change appearance, we gain, we lose weight, we put makeup, we do this, facial appearance can change a lot, Grow beard. Irish is very stable in that respect, and it's something you're less likely to mess with, surgically anyway. Right? Now, you can put lenses.
Marios Savvides:
Right? You can put, you know, those Halloween lenses to cover up your eye. But then, that's equivalent of me putting a bag over my my my my face and say, who am I? In the same way, iris. So you can detect if somebody is wearing it as putting a pattern over your iris because your pupil is even now as I'm talking to you, my pupil is is is dilating and contracting naturally. It's changing, and the iris pattern is also equivalently. In fact, and the way the iris pattern actually moves is it's in a circularly way. So if you ever seen those old series Stargate SG 1, you know how the iris there opens up the portal, it's kind of like that way your iris sort of rotates and and opens up and closes. And so, you can look at those you can look at those movements and see, well, is this somebody putting a fake lens and a fake iris? It's not gonna move that way naturally. So it's a lot harder to spoof.
Marios Savvides:
It's less to change. So, you know, if you literally, even from a baby, you know, it can drive your condition. So, yeah, I I love iris in that respect. Now the challenge with Iris is it's hard to capture. You know? And I apologize. I think my dog's snoring. Benjie, wake up. He always does this in
Vince Spina:
we love dogs, we love all dogs on this show.
Marios Savvides:
Yeah. He always does this in all my Zoom calls. And I said, guys, apologies. It's not me. I'm not snoring. It's my dog.
Vince Spina:
And we're not either. This is super interesting. So
Marios Savvides:
it's all good.
Vince Spina:
Benji's like, I've heard this before, Mario. Just keep going. And then he knows
Marios Savvides:
at the end, when he he knows exactly when I'm ending, he's just like wakes up, okay. Okay, dad. Should we go outside? I need to do an exhibit. Sorry about that. Okay. So where was I? Irises. So the challenge with irises is, how do you capture an iris that, you know, in the old days, you had this picture of, like, you're putting an eye somewhere, like, in this hole, and, like, you know, you felt like it was very, very intrusive. Right? It's almost like think of Minority Report, very intrusive.
Marios Savvides:
Although the beginning part of Minority Report, when Tom Cruise walks into the gap and he's recognized about a couple of meters away, that's pretty cool. Right? Well, most iris systems are anywhere between maybe 10, 30 centimeters, maybe some up to a meter, somewhere there. We've actually built a system now 13 years ago. Back in 2009, we started, we finished 2011. We built the world's first system to capture an iris 13 meters away, 40 feet. Wow.
Rachael Lyon:
I saw that video. It was kind of a long range. Right? Yes. Iris recognition.
Marios Savvides:
Wow. We built the world's first longest range iris system back in 2010, and it was and it's no. It wasn't x marks the spot. It was anywhere between 6 meters to 13 meters, and I could enroll someone. It wasn't just matching, I could actually enroll someone. So, iris to me is very fascinating, in that respect. I think it's one of the most robust biometrics that, you know, you can't fool, You're less likely, someone is less likely to go and mess with. And again, it's thought to be safe.
Marios Savvides:
The challenge with it is exactly that, capturing it in a way, in a long range way. And you know, when people say, oh, Irish recognition, long range, 30 meters. Oh, that sounds scary. That sounds intrusive. And I say, no. It's, you know, Irish recognition, fissure condition, The way I look at all of this is, it's human robot interaction. I have a computer system that is recognizing who it's interacting with. It needs to know.
Marios Savvides:
Right? Is it Marius he's talking to? Is it Rachel? Is it Vince? Who is it interacting with? So it knows how to answer. Right? If I step in the scene, it will continue a different conversation. Right? Well, that's how that's why that's how facial recognition came into play. It wasn't big brother or any of these things. It was humans and computer systems need to know who they're interacting with. And so, all we've done is make systems better at understanding who you're talking to by looking at their face or iris. And if you can do it in a way that's not intrusive because imagine, if you have to go and place your eye or do something that in any way goes out of your way to it's an explicit step, then it becomes intrusive. Then I feel that I'm getting my biometric captured.
Rachael Lyon:
Right. Right.
Marios Savvides:
Right? If I don't have to do anything, then I don't feel I don't feel anything happen. I don't feel it's an extra step. And that's why fingerprints always have the biggest bad rep because you're like going and you're putting your thumb or your finger on something and it just feels very abnormal. It feels extremely intrusive. Right? Now, they've gotten better. There are touchless systems now where you can just swipe through and it will capture your fingers, your fingerprints. And that's what I love about AI, the ability of building computer vision systems that can do that that fast, less intrusive, in my mind, removes some of the negative stigma that Hollywood has tainted and really, really stained in us. That it's, you know, I'm trying to remove the I really feel like one of those painters.
Marios Savvides:
I'm trying to remove the stain from people. Okay. Biometrics is not bad. Okay. It's not the root of all evil. You know, anything can be used for good or bad, but there's actually a lot of good. And we want system to understand who they're talking to. We we want a robot to know who to interact with.
Marios Savvides:
It it's just a more natural experience.
Rachael Lyon:
Definitely. And I hate to do this but we are at the end of today's podcast. We're gonna pick back up for part 2 next week. To all of our listeners out there, thank you so much for joining this week. And for our new listeners, welcome. If you're enjoying the conversation, please subscribe. We're on all major podcast platforms. Until next week, everyone.
Rachael Lyon:
Stay secure. Thanks for joining us on the To the Point cybersecurity podcast brought to you by Forcepoint. For more information and show notes from today's episode, please visit www.forcepoint.com/podcast. And don't forget to subscribe and leave a review on Apple Podcasts or Google Podcasts.
About Our Guest
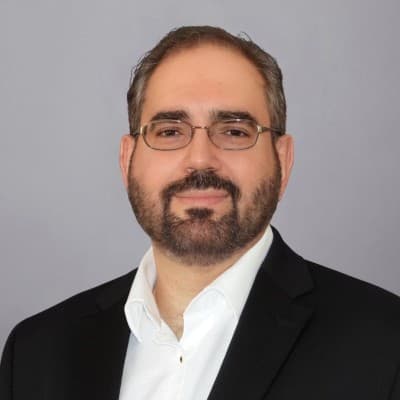
Marios Savvides, Director of CMU CyLab Biometrics Center, Carnegie Mellon University
Professor Marios Savvides is the Bossa Nova Robotics Professor of Artificial Intelligence at Carnegie Mellon University and is also the Founder and Director of the Biometrics Center at Carnegie Mellon University and a Full Tenured Professor in the Electrical and Computer Engineering Department. He received his Bachelor of Engineering in Microelectronics Systems Engineering from University of Manchester Institute of Science and Technology in 1997 in the United Kingdom, his Master of Science in Robotics from the Robotics Institute in 2000 and his PhD from the Electrical and Computer Engineering department at CMU in 2004.
His research is focused on developing core AI and machine-learning algorithms that were successfully applied for robust face detection, face recognition, iris biometrics, and most recently, general object detection and scene understanding. He and his team were the first in the world to develop a long-range iris capture and matching system capable of acquiring irises up to 12m away in an unconstrained manner. His recent work includes ranking first in Vision for Intelligent Vehicles and Applications competition for hand detection on steering wheels in natural challenging driving conditions. Some of his recent work can detect heavily occluded faces and objects in general under very challenging real-world conditions, developing low-shot object detection and recognition utilizing only a small number of images.
Professor Savvides spun off a CMU startup called HawXeye with one of his former students where he served as CTO. As the CTO, he assembled a team and lead the research and productization of efficient, fast, low-form factor AI algorithms making current generation of home security cameras smarter where the AI algorithms developed have been deployed to over 3 million ADT home security cameras with a successful exit.
In the last 24 months, he served as the Chief AI Scientist of Bossa Nova Robotics, where he and his CMU research team, completely re-built from ground-up the AI algorithms for Bossa Nova robots for performing real-time inventory analysis and scaling the autonomous robot deployment of this inventory analysis AI from 20 stores to deploying 500 autonomous robots in 500 retail stores while completely removing any Human-in-the-Loop (HITL).
He served as the Vice President of Education for the IEEE Biometric Council in 2015-2016. He also served on the main steering committee and helped co-develop the IEEE Certified Biometrics Professional program.
He has authored and co-authored over 240 journal and conference publications, including 22 book chapters and served as the area editor of the Springer's Encyclopedia of Biometrics. His IP portfolio includes over 40 filed patent applications with 15 issued patents. He is the recipient of seven Best Paper awards. His work in facial recognition was presented at the World Economic Forum in Davos, Switzerland in January 2018. His work has been featured in over 100 news media articles. He is the recipient of CMU’s 2009 Carnegie Institute of Technology (CIT) Outstanding Research Award, the Gold Award in the 2015 Edison Awards in Applied Technologies for his biometrics work, 2018 Global Pittsburgh Immigrant Entrepreneur Award in Technological Innovation, the 2020 Artificial Intelligence Excellence Award in “Theory of Mind”, the Gold Award in 2020 Edison Awards for Retail Innovations on Autonomous Data Capture and Analysis of On-Shelf Inventory, and the “2020 Outstanding Contributor to AI” award from the US Secretary of the Army Mr. Ryan McCarthy.