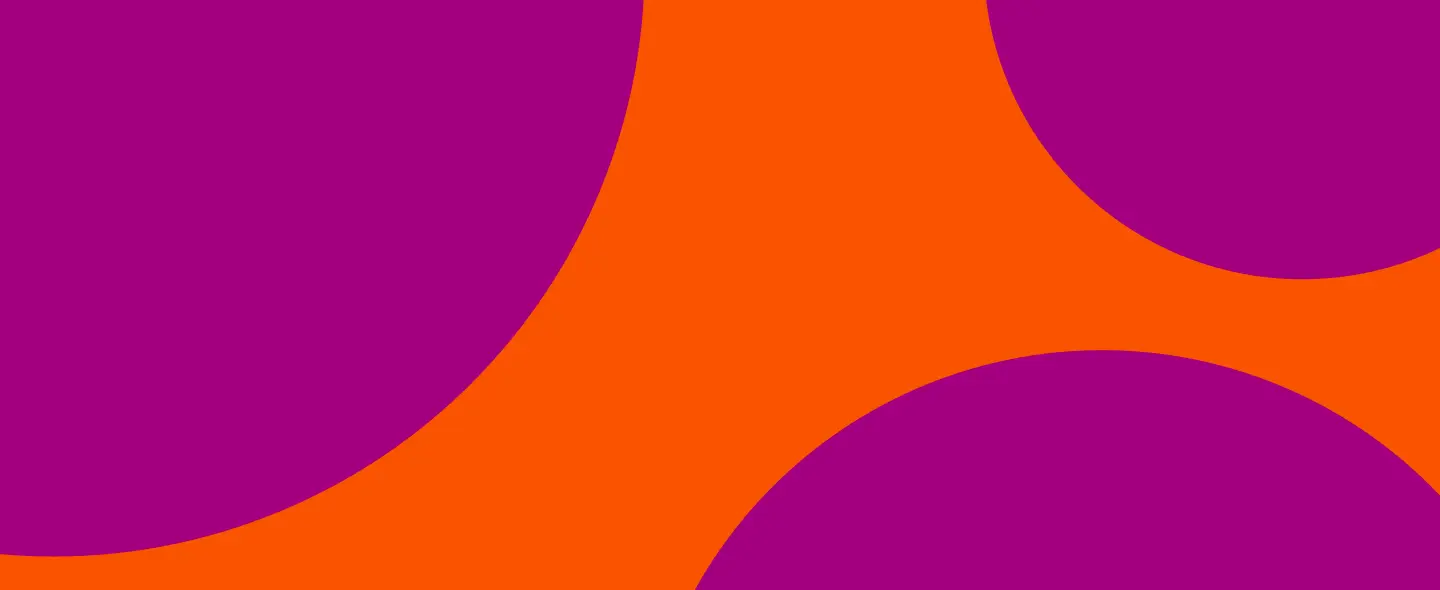
Back from the Archives: It's All in the (Deepfake) Experience
Share
Podcast
About This Episode
Back from the archives: This week, we're replaying a popular episode with Dr. Siwei Lyu, a SUNY Empire Innovation Professor and founding Co-Director of Center for Information Integrity (CII) at the University at Buffalo, State University of New York. Siwei breaks down the deepfake experience, both the good and the misleading aspects of the technology.
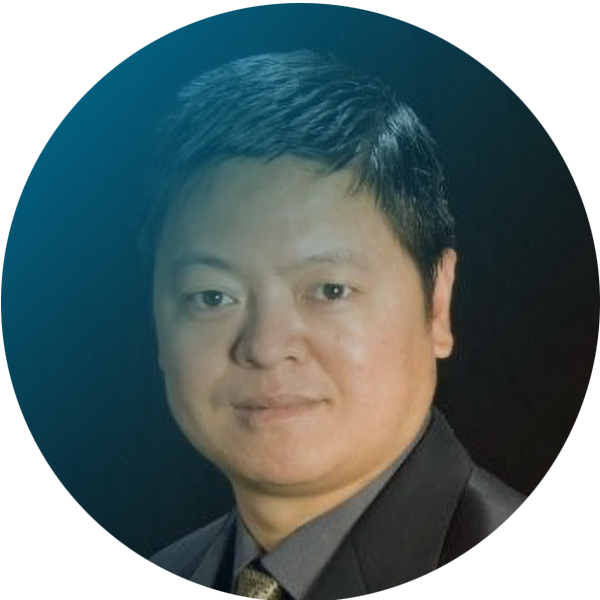
He shares insights on techniques researchers are developing to detect deepfakes, including GAN (Generative Adversarial Network) generated artifacts that produce tell-tale deepfake signs – if you know where to look. He also delves into the area of audio deepfakes and the sophistication of the human auditory system that makes this pathway a tough one to win. And, fun fact, to learn more about Siwei’s research contributions be sure to Google “DeepFake-o-meter!”
Podcast
Popular Episodes
Podcast
Back from the Archives: It's All in the (Deepfake) Experience
[01:20] How Dr. Siwei Lyu Found His Way to Deepfakes
Rachael: We've got Dr. Siwei Lyu. He's a SUNY Empire Innovation Professor at the Department of Computer Science and Engineering. He's the Director of UB Media Forensic Lab, and the founding co-director of Center for Information Integrity at the University of Buffalo, State University of New York. Welcome.
Eric: Thanks for joining us, Dr. Lyu. Rachael, cut your bio by about two-thirds of it out. If we read the whole thing, we'd be here for 20 minutes. You are quite accomplished in this space.
Rachael: He's so accomplished. It's kind of amazing. And you're like in a really hot area, the whole deepfake thing. And I saw your DeepFake-o-meter. I mean, there's so much we have to talk about today. I don't know if we have enough time.
Eric: The DeepFake-o-meter is the best.
Rachael: The best. I guess, let's start at the beginning. How did you find your way to deepfakes?
Siwei: Yes, it's quite interesting actually. I started working my PhD degree starting 2001, working this area called multimedia forensics. So like in the old days, multimedia forensics, meaning we want to detect if somebody Photoshopped an image or digitally beautify a face, make it look nicer. Those are serious problems. But relatively speaking, those are low tech and it's only like a few wheeze in your lung, that kind of thing.
Kind of you know their existence, everybody know they're bad, but nobody really take this seriously. But what is kind of interesting is another research interest, branch of my research interest is in artificial intelligence, more particularly in computer vision and machine learning. So those are core areas behind those AI algorithms. And on that side, I'm trying to use algorithm to do a lot of interesting things.
What Is Deepfake?
Siwei: So it was actually in early 2018, I was in a technical conference, I met with a colleague.
And he mentioned to me this thing called deepfakes. I was very intrigued by what it is. He told me it was basically somebody using an AI algorithm, create a fake video and spread it online. And a lot of people seem to be fooled by it.
It's almost like a magical moment because he just point the two major interests of my research into one single point. In the past, I've been working on media forensics, algorithms, but not particularly using a lot of AI algorithms. On the other hand, I'm working on AI algorithms, but doing totally different things. And now here is a chance that I can combine both of them.
Also after digging in a little bit more, I sort of feel like the potential significant negative impact this kind of technology can have to the society. And I think it's that moment I got both scientifically curious about this topic and also socially aware that the impact this can have to us, to everyone. So I feel like this is going to be a very interesting research topic to investigate and have my student working on this. And in about three, four months we come up with our first method and everything start from there.
Eric: I feel like deepfakes, a lot of people talk about them, they have a high-level understanding of what a deepfake is. But I have yet to run into many people who actually think about it in their day-to-day business or what they're doing.
AI Synthesized Media
Rachael: They don't look at some YouTube video or some Twitter video or some TikTok, whatever it may be, and really question, "Did Nancy Pelosi really say that?" Or, "Is that a deepfake?" Just in my travels and speaking with people, they're aware of deepfake, Dr. Lyu, they don't seem to apply that awareness and practice in day-to-day practice. Are you seeing the same thing?
Siwei: Yes. Initially at least. Right now, I think a lot of people talk about deepfakes and they become more aware of it. Just like one little note, the Nancy Pelosi video, where we saw, I think two years ago, become viral on YouTube, that was actually not a deepfake. People called it shallow fake or cheap fake. The way it was created was actually by slowing down the playback speed of that video. So make her sound like slow-talking a little bit sloppy and those kind of feelings. But no AI algorithm is used and no content was actually changed. It was just a presentation of the content.
So this comes back to the definition of deepfakes and what we mean by deepfakes. It's basically a street name. I usually use the name AI synthesized media to make it sound more neutral. Deepfakes necessarily carry this inactive feeling with it. But what do we mean by deepfakes using that term, is to refer all forms of audio, visual media that are either synthesized completely or manipulated with the help of AI and particularly something we call deep learning technologies. Deep learning is a special type of technology in AI. So you combine the term deep learning and fake media to coin the term deepfakes. So that's basically the origin of this kind of things.
Robert Pattinson’s Deepfake Experience
Siwei: I think people are becoming more and more aware of deepfakes these days simply because it's so up many times in media. We are seeing a lot of examples emerging on all the social platforms. I can count my finger for some of the most recent examples. Very recently there's this synthesized video of Ukrainian president, Zelensky. Talking about all the European citizens putting down their weapons and surrendering to Russia.
Two days ago, I was interviewed by MSNBC. They found out a fake TikTok account impersonating actor Robert Pattinson. It was a viral video because it looked so similar to the real person. You've got millions of views and spread it fast online. So I think as we're seeing more and more of these examples, people are becoming more aware of it.
Eric: They're aware that they're out there? Or they're aware that what they're watching could be a deepfake and could be influencing their perceptions of reality?
Siwei: I think mostly the former, they're aware of the existence of deepfakes. But we actually did a study, do a survey of some online users. We found out almost everybody know the existence of deepfake. But when we actually ask them, "Have you ever seen a deepfake without your awareness. Not something pointing to this as a deepfake and look at that, but something you figure out as a deepfake." Almost nobody said they have seen it.
So I think we're talking about two levels of awareness. One is the awareness of their existence. The other is the awareness of the closeness of that existence to everyone of us. I have to say there are a couple of reasons for this. I think one particular one is the deepfakes we're seeing now are mostly making for fun or exaggeration.
President Zelensky’s Deepfake Experience
Siwei: So for whatever reason, we haven't seen a deepfake video that is sneaking in that look realistic enough, but claiming a message, not so ridiculous or outreaches. That's societal but it's misleading. I think that kind of deepfakes will really cause concerns. But for some reason we haven't seen that. I think technically that's already possible, we're just not seeing them many examples at this moment. But that doesn't speak for the future.
Eric: Is there any research out there showing what percentage of the population, wherever that may be, has been exposed to a deepfake? Like, I don't know that I've seen one that influenced my decision-making process, but I don't know that I haven't either.
So a lot of us saw the president Zelensky deepfake. But I wasn't laying down arms and I think it was so broadly spread that, I'm not in the Ukraine. I think it was so widely spread. Media covered it. You know it wasn't legit. But I don't know if there are any others that impacted me per se, or Rachael maybe, that we aren't aware of, but it did influence our thinking. Have there been any studies?
Siwei: Not particularly. I think the study is fairly difficult to conduct.
Eric: Right. How are you going to do it?
Siwei: The other thing is, if you're not aware if something is a deep deepfake, it's very hard to say, "I have seen a deepfake."
Eric: That's the problem with this, this disinformation, Rachael, you can't find it.
Siwei: Well, there's other causes of this problem because, one, I think one of the dominating negative use of deepfake technology is pornography. And especially this is called revenge pornography.
[12:07] Why People Watch Deepfake
Siwei: So after a breakup, a former spouse or boyfriend, usually the victims are women and they use the access to the footage. The private footage of the victim and make a pornographic video or image and plant the victim's face into it.
I was actually involved in one of the legal cases as an expert witness. And what I learned is many cases, for that particular case, it doesn't matter if the video looks real or not. Sometimes some of the videos are made very crudely, reasonable artifacts is all over the place.
There's no difficulty for anybody to tell this is a fake video, but you still get a lot of people viewing it. So I think humans are very complicated in this sense. Because we go online searching for information, not just for knowing the truth, but a lot of time with other kind of intentions. And sometimes we're just looking for the most eye-catching, the most sensational, the most interesting, exciting.
That usually is not the true information because the truth is boring. So that's why most known deepfakes are in that general. It got a lot of people's attention because it's showing something abnormal, out of the normal, and interesting. There are some study about how many deepfakes we know existing online. But that's only the tip of the iceberg, and nobody really know how many we actually have.
Rachael: Right. I mean, how could you know? I was reading an article and I think it was from March last year where you were talking about one way to spot a deepfake is in the eyes. Something about the reflection in the eyes.
We’re Dealing with Algorithms When Handling Deepfake Experience
Rachael: Could you talk a little bit about that? And is that still the case? Or has the technology already evolved so quickly that that's not a tell sign anymore?
Siwei: I will answer the second part of the question first and then talk about that work. This is actually a nature of this line of work I learned throughout 20 years working in this area. Unlike other scientific research fields where you deal with a natural phenomenon, something doesn't change. It's very nature, complicated, difficult to understand, but it's a fixed problem and you just tackle it.
For this line of work, it's more like a cybersecurity. We're basically dealing with algorithms. So the problem is by itself is very dynamic. And on top of that the current scientific research model is we develop some methods, we publish in the paper, we basically serve this with everyone. So algorithms can take advantage of that and improve their algorithm.
So I think it is always a cat and mouse game. And I think in this case, it's a very tiny cat with a big mouse. So it's a kind of competition or arms race that we are sort of on the losing end because of that. And just think about how much incentive for those people making deepfakes in comparison with we trying to expose them.
I don't want to be over pessimistic, I think we're catching up, but it's always dynamic. Whenever we figure out something, I'm sure at certain stage, at certain point someone will be able to fix their models and remove that. So coming back to this, I mean, that's something we observed and it's particularly applicable for synthetic human faces. So there's a model known as generative adversarial network.
[16:24] Generative Adversarial Network
Siwei: We usually use the short name GAN for it. And the GAN models have been shown to have the ability to create highly realistic human faces. And if you put them side on side, it's very, very hard to tell apart from real human faces and those synthetic faces. What is more important is, there's a recent study showing to human subjects, those synthetic faces and real human faces. And turns out that most of the human viewers view the synthetic faces as more trusting.
That's why those online trolls, they set up fake social accounts on LinkedIn, Twitter, and Facebook, instead of stealing somebody else's images use as a photograph. They now use GAN-generated images. Simply because it's harder to trace and they also look better. So this is the problem we have to deal with if we want to tell if an image is a real face or is generated by this model.
So the model is quite powerful. Being able to create all those high fine details in hair, on the skin, and so on. But we also figure out that the model has an Achilles' heel. And this is kind of interesting observation. It's kind of like we have an aha moment. We have enough number of this kind of images. And I stare into those images, there's always something kind of funny looking at them. They all look real. And then one day I realized that the true eyes, when you look at the reflections. Our eyes is a very amazing organ on human body. It's the only one that have a regular geometric shape.
How to Spot Deepfakes
Siwei: So the iris part is almost close to a perfect sphere. And not only that, it's like a mirror. It's almost like a perfect mirror. It reflects lights very effectively. So if somebody's taking a photograph, closeup portrait photograph of us, if there's anything in the scene that either emitting light or reflecting light, like, Rachael, in your room right now there's a light there. And that light, when it reaches your eyes, it will leave an image. That image is a reflection.
Of course, in comparison with the size of the room, the distance between our eyes is very small. And the eyes are, if we assume roughly the two eyes are in the same plan, then they are roughly looking at the same things. So very intuitive, visually each packed. Whatever the two eyes see are roughly the same.
That boils down to, if we look at the reflections, the highlights, the specular reflections, they should also be similar. Because the two eyes are essentially looking at the same scene at the same time. But when we look at the GAN-generated images, it's different. So even those GAN models are very powerful, capturing all this nuance of human faces. It has trouble understanding this basic physics. And it's not rocket science. It's very simple piece of physical constraint that the two eyes need to look at the same thing.
So when we look at the reflections from the two eyes of GAN-generated images, they are very different. And almost like the two eyes are looking at two different scenes at the same time.
Eric: That should be easy to fix. Shouldn't that be easy to fix?
Why Fixing the Images in the Eyes Is Not the Perfect Fix
Siwei: It can be fixed easily by just copying one eye to the other, but there are two reasons that is not a perfect fix. One, the two eyes, the two images are, as I said, they're similar but they're not exactly the same. So if you just copy-paste, we can do all the geometric analysis and you can see that they shouldn't be exactly the same. There should be a slight shift and we can calculate that shift and we can know if they are exactly the same copy and that's equally unlikely.
Eric: So that's one of the ways your software can determine whether it's real or a deepfake?
Siwei: Yes. We use that as a shield. And I think the kind of advantage of this cue is very intuitive. I can explain this to everyone and they immediately understand why we make that decision. But on the fixing side, so that simple fix works to a certain extent, but cannot fool this algorithm.
But they do, can fix this by giving a lot more data, and specifically with data about the reflections to be consistent on both eyes. And I have to say all this deepfake generation models, they are as smart as the data we feed them. So the first round, we are able to capture these artifacts because the first round of generation model, whoever trained these models are not careful enough to put data, specifically emphasize, putting this kind of data there.
They actually need a lot more data to make this constraint to happen coherently. So it was fixed. I think this new run, there's a new, very recent model coming from the second generation. We now have the third generation of that, GAN generated images.
Look for Those Achilles’ Heels of the Models
Siwei: Because I see enough number of those images, I can still see slight off of those reflections. But roughly when you look at them without too much attention, they look pretty realistic now. So that is one that's the very nature of this problem. But what are we trying to do here is always look for those Achilles' heels of the models.
After the discovery of this reflection pattern, we have also identified the shape of the iris. Iris is the central circular opening of our eyes. And for healthy adults, the circle of iris is almost perfectly circular. They look like a circle. In a GAN-generated images we have an algorithm that can automatically track iris shape, and we can compare that with a perfect circle. It turns out that the second generation of the GAN models do not have a good circular shape. And that is also a very strong cue. But we need to look into those details where the model ignores, and we can pick it up, use as a detection.
So we are playing a lot of detective work, but we translate that detection, the intuition for detection into algorithms. So we want to do this automatically with an algorithm people can use, instead of asking people. Of course, a lot of people after they read the paper, they start to pay attention to the eye region. But I think as a researcher, our ultimate goal is you have to either incorporate all these kind of cues into an automatic detection algorithm.
The Algorithm Can Analyze What the Naked Eyes Cannot See
Eric: But it's got to be hard if you're watching a video on your iPhone where you can't see the eyes very clearly. They're small and you just see it and believe it. And I've got to believe that's one of the bigger consumption devices people use these days, whether they're on YouTube or Facebook or wherever. How hard is the audio? I'm assuming that's easier than the video to fake.
Siwei: Actually not. I was surprised by this fact. But let me say a little bit about when those media are shown on small screens. So using this kind of physiological or physical cues is only one approach we rely on to detect deepfakes. We have another approach which is looking for something that is truly invisible to human eyes but only visible to algorithms. So you can sort of think about this in the analogy of the x-ray. X-ray shadow on human body will help us to see something otherwise naked eyes cannot see. So we have algorithm looking for signal signatures from those deepfake media.
When they were generated, fundamentally they are different from real media. And real media come from a camera or a microphone, capturing a physical event in the physical world actually happen. But this kind of media is created using an algorithm completely out of the vacuum and is digital. So that fundamental difference actually will land in some differences at the signals, when we look at them as a sequence of numbers. Those differences are not as intuitive as the eye reflection or iris but nevertheless, we can have algorithm. If we train the algorithm on a large set of fake media and real media, we could have some approach.
[26:16] Audio Synthesis Is Harder to Figure Out Than Images
Siwei: Some level of confidence to pick out fake media that have similar signal signatures as things we have seen previously. So I think the answer to Eric's question is we have other approaches complimentary to what we have described. And in this area, it is always like in the pharmaceutical industry, no medicine's going to be the magical medicine, we have to have a compound solution to this.
Now, coming back to the topic about audio, it's very interesting that audio synthesis is actually harder than images. I think the fundamental reason is not really too much of the technical but mostly because our audio system, human auditory system is very different from our visual system. Our auditory system is particularly sensitive to artifacts. So if there's anything that is a little bit off, our ear is very sensitive to that. On the other hand, if we have an image, have a few pixels off, our eyes are not very good at picking those artifacts to a certain extent.
So the audio, if we have a little bit like noises, like those kind of abrupt abnormal songs in those audios, we can pick it up easily with our ears. But I have to say, at this moment, the audio synthesis algorithms are catching up. In my lab, we have examples where we can generate voices of celebrities like President Obama or President Biden using algorithms. If you listen to them very carefully, you can still pick up those artifacts. But if you just listen to them once, I will say for casual listener, those artifacts can just go under the radar.
Local Phases
Siwei: We also have an audio detection algorithms of audio deepfakes. And that is again, based on some interesting physical observations. It is through this line of work, I have an in-depth study of human auditory system and finding it absolutely amazing. One thing we probably are not aware of is the fact that our ears have this amazing ability to discount something we call local phases.
So let me expand what this means. While we were talking here, suppose we were talking face to face in a room. What happened is my mouth is making the sound. And that sound is a wave and transmitting in this space and reach your ear and you hear my voice. But it actually is not that simple because when I'm creating the sound, the sound wave does not go into one single cast, starting from the mouth through our ear, actually goes everywhere. So it goes to the wall, goes to the ceiling and it bounces back. And this is how the audio engineers mixing the songs. They won't have all the songs kind of mixing together.
Very simple phases showing that if you are hearing the sound, you are actually getting multiple copies of my voice from different passes. And this different copies, even though they're the same sound, they arrive at your ear with slightly different time. Because they go through different passes. If it go straight line or go to the sitting, and then passes.
Eric: You get a lot of reflection.
Siwei: Yes, you got reflection, you get multiple passes. And sound go in air with the fixed speed. So that means they will reach your ear at a different time. And that different time in signal we call local phases. There's a difference in local phases.
The Interesting Capability of the Human Auditory System
Siwei: So our auditory system have this very interesting property of discounting that local phase differences. It's important because otherwise, and we actually know there are known cases of patients who due to genetic defect doesn't have disability. So they cannot hear anybody talking in a stable way. Because all they're hearing is this harsh parts of sounds mixing at different timing. It's almost like you're in a room listening to multiple people talking at the same time. It's very hard to find out that particular message.
Our ear can do this very adeptly. When the arrival time is truly very different, like in the echo, you sat in a valley, there's echo. The sounds coming back at a very different time, now you hear two voices instead of one. So it's very amazing. And at this point we don't really know the biological reason how this is here.
We actually take that as a hint to analyze local phases. Because all we hear, our ears or our brain discount those differences in local phases and then somehow combine them into a single signal. And we are only sensitive to the amplitude or the energy. That's how you can hear me speaking. Whatever comes to our ears that have the same energy profile, we treat that as a good sound.
There are AI synthesized audios. When we listen to them, they are very natural, but we take this as a hint. We look into the local phases since our ears are not sensitive to local phases. When those algorithm generates those voices, they don't care about how they're going to arrange locals.
How Easy Is It to Create Deepfake?
Siwei: Most likely the local phases of a real sound is completely random because it goes through different passes. But those algorithms, your local phases has a very specific relations. So you can identify those kind of structures in those phases that shouldn't show up in natural voices. And then we use that hint and device an algorithm, again, like an x-ray. You look into this direction where we will not be able to hear or see with the waveform. But we can capture that difference in local phases. And that is an algorithm we developed in 2018.
Eric: So you've got all these techniques to detect deepfakes. How easy is it to make them?
Siwei: You mean developing the method or how easy to make deepfakes?
Eric: No, just a user, somebody wants to create a deepfake.
Siwei: I think that's actually one of the major reasons why deepfake is dangerous. It is not like we cannot make face modeling videos, realistic human faces, or voices 10 years or even 20 years ago. If we watch Hollywood movie, we see all those special effects, blue screens, voice-over, we already have the capacity of making them. But the problem is those are for people with special training, special equipment, big budgets. What deepfake change is now you can make them, everybody can make them. All you need is a computer, internet, a fast computer internet, and a large hard drive. And the algorithm, many of the algorithms are freely available online. Somebody may even package them as apps on your cell phone. So the democratization of this capacity is what makes us concerned.
[34:45] DeepFake-o-meter
Siwei: So I think that's really the problem. These days I think audios are a little lagging behind, but there are several startups providing this as services. For face locking videos there are apps and well-packaged softwares. Also open source code on GitHub, and everybody can download them and can use them. So it's becoming easier and easier.
Eric: So I can do it myself, I can outsource it, pretty easy to get. And the other thing, it doesn't have to be a hundred percent. I mean, if you detect a deepfake here, I can try again and again.
Siwei: That's right. Again, as I said, this is dynamic, so it's always a back and forth.
Eric: Another problem on the show, Rachael, we can't fix today.
Rachael: Well, I know we're coming up on time here, so I did want to kind of close out our last question so you could share with our listeners about your DeepFake-o-meter, which I believe has a very high success rate? In detecting deepfakes.
Siwei: Yes. Well, I will say the DeepFake-o-meter is our effort to bundle all the existing deepfake detection technology and make them usable for the users. Unlike making deepfakes, detecting deepfakes, there's really a vacuum of services provided in this way. On the other hand, there's a lot of research also for detection algorithms. But those research are usually code on GitHub. And for somebody who just wants to analyze an image or video, they need to know how to set up the environment, download the code, to compile them, and run them. So all those are actually a hurdle for user to take advantage of those detection algorithms. So that's the DeepFake-o-meter.
Check the Deepfake-O-Meter Tutorial in YouTube
Siwei: So we didn't develop all the detection algorithm, although we have a few in there. But what we're trying to do here is actually collecting known open source detection methods and put them in this platform. So users can just pick and select a subset and run on their media. So that's what we're trying to do. It got a lot of users but also being abused I will say.
We have several times got hacked, people trying to do denial of service. Or we have seen abnormal trolls of users trying to run algorithms. I think sometimes they just want to test out the departures so that they have a very high frequency of trying it out and getting the results. These are all the things that were unexpected to us. So there's a lot of, I would say interesting experience in setting up the platform. Right now, the platform is in this stabilizing stage, and we're trying to augment it with more detection algorithms. So we are looking online and whenever there's a code that we can incorporate, we'll look into that.
Rachael: Very cool. And I highly recommend everybody go to YouTube and look at the DeepFake-o-meter tutorial. Because it's really cool and so easy to use if you want to try to see if you've got a deepfake on your hands.
Eric: What does the future look like? I mean, is it really some kind of AI that can think and respond and look and sound like you, but it's not you? Is that where this ends up eventually? Or what does the future look like in your opinion?
Rachael: Like your avatar, like your deepfake avatar?
The Future of Deepfake
Siwei: Yes. That's certainly probably the future. I cannot tell the future, but it does seem that we are approaching toward that direction, let me say it this way. But I think the future of deepfakes is we're going to see more realistic synthetic media being made more cheaply and easily. And also not only things like faces or voices are being fabricated. We're also going to see natural scenes, objects, cars, your pets, and also whole body, whole human body actions will be generated. And I think that trend will be unstoppable. People are trying out AI algorithm to meet up these challenges.
And actually, I want to say, although we think deepfakes mostly about their negative impacts, their existence is actually a tour of the front to the force of the AI technology. We can create realistic human faces is actually a huge achievement for AI researchers. So I think that ultimately will not stop.
But I would always say deepfakes coming off of algorithms will always carry some kind of artifacts. And it takes human in the loop to fix all those artifacts. So I think, I don't see humans going to completely disappear from this whole scene being replaced by our digital twins or digital existence in any near future. I think humans are going to play actually a more and more important role in this. But that's really what we have to be aware of and device some better detection models.
Eric: We learned so much. Good luck with the DeepFake-o-meter and fighting the good fight here.
Siwei: We'll keep doing that. We live and learn. A lot of lessons we're going to incorporate into our next installation of the system and making it better, make it easier to use for the users. So thank you very much for your interest and this is a great conversation.
Rachael: Thank you. And to all our listeners out there, thanks for joining us yet again for our weekly podcast. Aagain, don't forget you smash that subscription button and you get a new, fresh piping hot episode delivered straight to your email every Tuesday.
About Our Guest
Dr. Siwei Lyu received his B.S. degree (Information Science) in 1997 and his M.S. degree (Computer Science) in 2000, both from Peking University, China. He received his Ph.D. degree in Computer Science from Dartmouth College in 2005. From 1998 to 2000, he worked at the Founder Research and Development Center (Beijing, China) as a Software Engineer.
And from 2000 to 2001, he worked at Microsoft Research Asia (then Microsoft Research China) as an Assistant Researcher. From 2005 to 2008, he was a Post-Doctoral Research Associate at the Howard Hughes Medical Institute and the Center for Neural Science of New York University. Starting in 2008, he is an Assistant Professor at the Computer Science Department of the University at Albany, State University of New York.