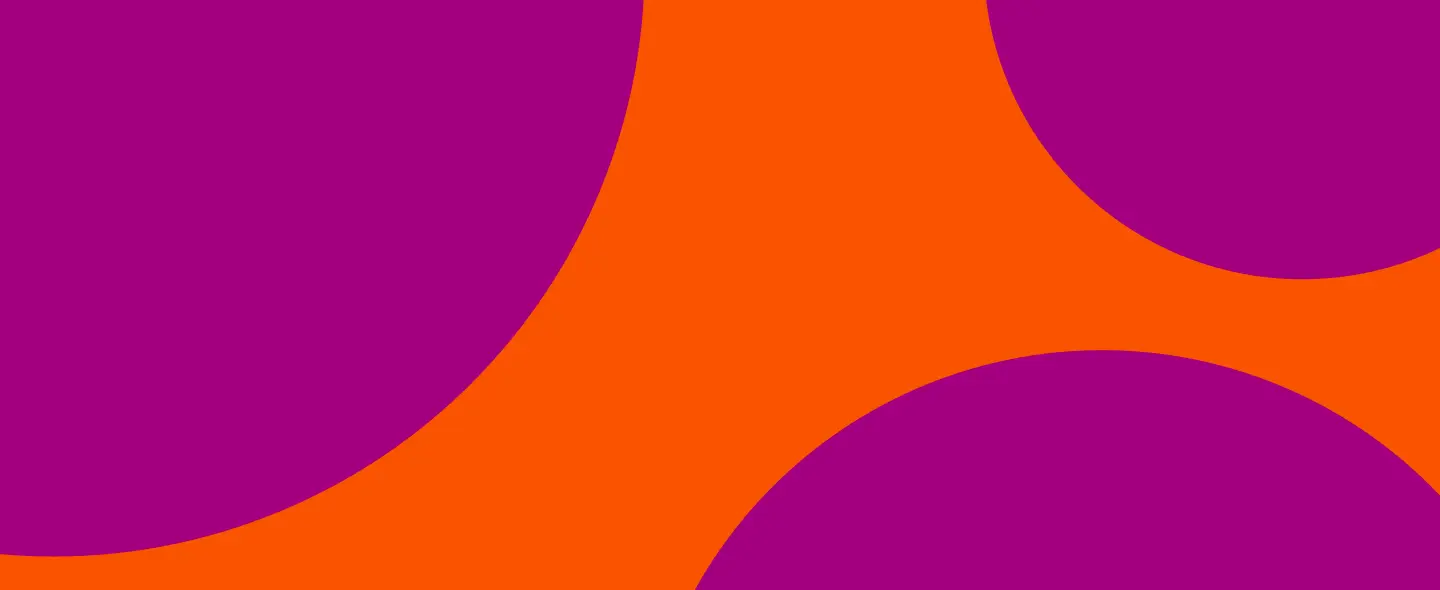
Use of AI for Cybersecurity with DHS' Martin Stanley
Share
Podcast
Popular Episodes
Podcast
Use of AI for Cybersecurity with DHS' Martin Stanley
What AI means for Government, where we are now, where we are going.
Episode Table of Contents
- [02:10] Different Ways of Describing AI for Cybersecurity
- [07:14] The Natural Relationship of Artificial Intelligence and Cybersecurity
- [12:01] An Evolving Attack Surface
- [18:41] Expanding the Aperture Around AI for Cybersecurity
- [24:46] Choosing Places to Pilot AI for Cybersecurity
- About Our Guest
Different Ways of Describing AI for Cybersecurity
Arika: This week's guest we have Martin Stanley, who is the senior technical advisor at CISA, the Department of Homeland Security. How are you doing Martin?
Martin: Doing great. How's it going over there?
Arika: It's going great. We're all still under quarantine in our personal bunker.
Martin: Still working from remote home.
Arika: We're going to switch it up. We've talked the past few weeks a lot about just working from home, and what that means and cybersecurity, network security, all those things. We're not going to talk about that too much today. We're actually going to talk about AI.
Arika: Let's switch it up and do a little Sci-Fi. Martin, let's just start first with our listeners who may not be familiar with AI. What is it and how is government using it?
Martin: Artificial Intelligence, I don't think that there's an actual definition that everyone agrees on. Folks have lots of different ways of describing AI. What we're talking about generally are systems that are able to learn from data examples, generalize from data examples. That are able to leverage algorithms and to gain knowledge from data, is what we're really looking at.
Martin: Today the capabilities that we're seeing are what we call narrow AI which means they're very good at a particular purpose, classifying apples versus oranges when it looks at pictures. But if you would try to then have it determining what is a pear it might have problems with that because it hasn't been trained outside of that narrow application.
Artificial Intelligence and Machine Learning
Martin: Artificial general intelligence, which is the thing that we see in all the Sci-Fi movies, it seems pretty far off. It's obviously something that's a game-changer when it comes to all kinds of things. But we don't anticipate artificial general intelligence being here anywhere in the near term.
Eric: We've had a few people talking about artificial intelligence and machine learning. George Kamis the Forcepoint CTO. We had the CTO of Intel, Steve Orrin speaking a couple of weeks ago, a couple of months ago maybe now. How do you differentiate the two? I often hear customers using AI and machine learning very interchangeably, and I'm not sure that's appropriate.
Martin: Again, I think this gets down to those definitions. NIST actually released a, I'm looking over here on my other screen here. But the taxonomy and terminology of adversarial machine learning, which is an entire draft that's related to just determining what those terms are with respect to just that area of artificial intelligence.
Martin: We have these very different ways of understanding what these things are. As a consequence, we do have trouble talking about them as well. I like to back up when we talk about what is artificial intelligence and to talk a little bit more about the AI-related technologies that we don't talk about much when we talk about artificial intelligence but are so necessary in order to fully realize the benefit and potential of AI, such as IoT.
Looking More Into Bringing in AI for Cybersecurity
Martin: These are all these devices that we have out there that are going to have all these kinds of capabilities, different kinds of sensors, 5G, cloud, big data. These are all areas that are very interrelated and your IT monitorization approach is going to be critical to how you actually see the benefits of AI.
Arika: What are you most excited about in terms of when you look to the future? And just to your point, there's a realm of different things that may or may not happen depending on what happens in the future. What gets you excited when you think about AI?
Martin: What I think about when it comes to what's interesting to me about this, why did I shift from working exclusively in cybersecurity to look more at how we bring artificial intelligence in, specifically to support our cybersecurity programs? Is that it's so interesting the way that our entire workforce is going to have to transform, and how our entire work environment is going to have to transform.
Martin: Today we have subject matter experts that work on particular aspects in particular domains of expertise, with particular tools. Those work areas are going to have to be completely re-envisioned to take advantage of all these technologies. That means we're going to have to bring in folks with different kinds of skillsets.
Martin: All these areas that we just talked about, all of the related technologies. But we're also going to have to bring in human-machine teaming, AI safety, as well as all other kinds of things along those lines related to being able to do data analysis, measuring performance of the artificial intelligence systems.
The Natural Relationship of Artificial Intelligence and Cybersecurity
Martin: All those things we're going to have to be able to execute on in order to build the most important thing, which is trust. Obviously, if the systems are not trusted, if we don't feel good about what they're doing, then we're not going to be able to leverage them to their full benefit.
Eric: Martin, one of the things we've talked about here is the skills gap between the required jobs today in cybersecurity and the number of people that are available.
Eric: I know you've spoken quite a bit in the past about using artificial intelligence and technology to kind of, I don't want to put words in your mouth, but upscale the capabilities without throwing human bodies at the problem if you will. Can you elaborate a little bit there?
Martin: Artificial intelligence and cybersecurity have a natural relationship. We've been using narrow AI solutions for cybersecurity for a long time, like spam filtering, things like that. Those are generally narrow AI solutions that are in place. Cybersecurity is a big and evolving challenge obviously, that's one of the main focuses of our agency.
Martin: At the Cyber and Infrastructure Security Agency, we're focused on being the nation's risk manager and lead for cybersecurity information sharing among other things. In order to make the biggest impact that we can with the funds that we have, we have to look at all different ways that we can leverage automation in particular technical approaches. Whether they become more self-serve or they become faster in our ability to deliver our services.
The Biggest Promise for Automation
Martin: The more that we can get and squeeze out of the appropriated dollars for our agency, the more we can do to help to meet our mission and to protect the nation and our critical infrastructure from adversary.
Martin: This means that looking at all these tools and capabilities and if we can go from doing 30 assessments to doing 3000 assessments. Because we've been able to automate critical time-consuming areas that are repetitive and leave maybe the exceptions for the subject matter experts. It makes the work more interesting for our subject matter experts, and it makes our ability to make an impact that much greater.
Martin: That's probably the biggest promise for automation for us. In two areas, one, expanding our capability and also reducing our time to respond. Because those are the two dimensions that we have to be focused on. How do we support all of our stakeholders and how do we meet an adversary that has increasing capability and ability to move quicker with each advancement?
Eric: Let's talk about the adversary for a second. In your experience, do you find the adversary is using artificial intelligence against us as much as we're trying to use it to defend ourselves?
Martin: The answer to that question is somewhat complex and maybe unknown. We do understand the way that these tools can be used against us. In particular, I think things that we are concerned about are deep fakes. The ability to customize malware, so that then it eludes the malware detection systems. These are the capabilities that I think we're worried about as threats today.
Artificial Intelligence Technology From Three Perspectives
Martin: But the way that we think about it is a little more comprehensive. In the context of your question, let me back up and say, we look at emerging technologies. Things like artificial technology, artificial intelligence technology from three perspectives.
Martin: The first one is something that we've just been talking about, which is how can we enable our mission and how can we better make use of that appropriated dollar to have an impact across our stakeholders? I won't beat that one to death.
Martin: The second area is one that we really haven't talked about, which I think is very closely related to the question you've asked Eric. Which is how our stakeholders are going to be using these technologies and what kind of change to the attack surface do we now have?
Martin: When we talk about threats, that's based on a certain set of capabilities but all the while that the threats are evolving. Our stakeholders are evolving their infrastructure and they're adopting these technologies. That means that now we have to think about, well, when a stakeholder deploys an artificial intelligence system what kind of new ways can that be attacked?
Martin: And what different ways could that system potentially be malpurpose or interfered with in such a way that could impact our stakeholders in ways that we don't imagine? These are unknown impacts, the unknown unknowns as they're called.
Eric: Like if an adversary knows we're using artificial intelligence in a certain area, they might poison the model if you will by attacking in a non-logical approach. Because they know that there's some model running to throw the data off.
An Evolving Attack Surface
Martin: That's exactly the kind of thing that we have to now be prepared. When we're looking at the threats, it's not really we don't have a fixed attack surface. We have an evolving attack surface.
Martin: That's including these technologies. We have to be prepared as we move forward as we're looking at this holistically at that start area with how are threats evolving, how our adversary is going to be using these capabilities. But it's not in isolation to how things are evolving in the target networks or the stakeholders that we're supporting.
Martin: Because as they deploy these new capabilities, they may or may not know that they're introducing additional vulnerabilities or additional attack surface. It's coming upon us to do our best to make sure you stay up with that and to make them aware of what are the risks and what are the ways to mitigate those risks?
Arika: My question was going to be and to the degree that you can say, how good of a job are we doing in terms of keeping up with this? Again, the evolving technology and the new risks that it brings to us, especially as we do see the threat surface it's expanding.
Martin: It is, and I think we have the concerns and these aren't really areas that I'm an expert in. So I probably won't go too far into them other than to say that they're the things that we see all the time. There's the disinformation, misinformation campaigns, these are the election security things.
The Stakeholders’ Response on AI for Cybersecurity
Martin: All of those areas that we are worried about are square in this new technology being used in ways that we might not necessarily understand. How do we best respond to those? Those are the kinds of areas that we want to focus on providing solutions.
Eric: How's the reception from your stakeholders? We're talking the civilian agencies in most cases, probably commercial industry. What's the reception from them when you talk to them, are you talking about what's coming or how they need to change?
Martin: I think the federal government is actually doing an incredibly good and comprehensive job in the artificial intelligence lane. I think we've talked about some of the things that we've been involved in, but there was an executive order for artificial intelligence.
Martin: There's a National Security Commission for Artificial Intelligence which is they've issued their interim report and they are doing quarterly recommendations to Congress, based on the work that they're doing. This is chaired by Eric Schmidt and Bob Work, two very high-level luminaries in the artificial intelligence space. There's a lot that's going on at the high-level policy area.
Martin: But then also when you look at the work that the federal CIO is doing to establish a federal data strategy, which is critical. 80% of some artificial intelligence implementation efforts are related to data, and dealing with data. That's happening at the federal level. The federal CIO is working on that. Then NITRD has updated their artificial intelligence R&D roadmap. I think it was for 2020 or 2019 they just released an update.
Bringing It All Together
Martin: There's a lot going on. DHS is involved in all these activities. There's so much that's happening. I just want to really go back and emphasize that putting a lot of money and putting a lot of focus on AI without focusing on some of these other related technologies is not as effective. We won't have as effective a solution as if we have a full IT monitorization effort.
Eric: Bringing it all together essentially.
Eric: I was doing some research over the weekend, thinking about what I wanted to ask you. I had no idea according to Wikipedia, which isn't necessarily the most reputable place, but artificial intelligence was founded in 1955. It's been around a while.
Eric: Arika I'm not a mathematician here, but I think that's 65 years, right?
Arika: That's longer than I would have thought.
Eric: It was before really compute power existed and a lot of the components, Martin that you say are required to bring it all together. It's interesting that the discipline's been around so long.
Martin: Going back to those days, I think a lot of what was conceived or envisioned it's still not yet come to pass from a technical capability perspective. That's pretty interesting as well.
Arika: Martin do you watch Black Mirror? That's my other question.
Martin: I don't think I've ever watched Black Mirror, but I've watched a lot of things like that. I'll have to add it to my list.
Arika: While you're quarantined, it's a good thing for a tech person to watch in terms of what AI may look like in the future. Do you watch it, Eric?
Expanding the Aperture Around AI for Cybersecurity
Eric: I don't, I don't have the time. When I think about it, I think of simple things like C-3PO, R2-D2 where robots actually understand to some level what you're talking about. They're smarter than you. They're faster than humans. They aid the humans. At my age I guess that's my mindset if you will, into the art of the possible.
Martin: Well look at how complex just the autonomous vehicle thing has turned into.
Eric: Another great example yeah.
Martin: We thought that by now we would have vehicles that were driving us around with no problem, and it's a very hard problem.
Eric: It's interesting when you look at the vehicles and we'll pick on Tesla because I think they're one of the best out there. The studies I've seen have said we still don't have level five autonomous driving vehicles of course.
Eric: That doesn't exist yet. But when the driving system is engaged, even though we've had some mistakes, it's still a couple orders of magnitude more precise and better than a human driver.
Martin: Until it's not.
Eric: Until it's not. They have a mistake and boom you crashed. That's a problem. But when it's working, it's so much faster, so much better than the human driver. It just doesn't work all the time.
Eric: As I think through the problem set, it's like, okay, what we need to do is expand the aperture. Expand the capability of the environment, if you will. So that the artificial intelligence or capability we have in the autonomous driving is better.
Martin: I think that what works best and this is based on studies that have been done that who performs better, the human or the machine?
From a Culture of Ultra Safety
Martin: Determined by what that particular case happens to be as we just talked about. But what performs better in every case is a human-machine team.
Martin: That's I think where we really need to start to think about. It was very interesting short aside I read that the autonomous driving vehicles were ranked I think by Consumer Reports, I'm not sure it was Consumer Reports, but it was something like that. Volvo came in last for autonomous driving.
Martin: They interviewed one of the spokespeople for Volvo. The guy was like, "Look, we don't think autonomous vehicles are safe. Our systems are not designed to be autonomous, which is probably why we ranked last and we're okay with that."
Eric: They come from a culture of ultra safety. They invented the seatbelt and then they open-sourced they gave it to everybody to make the world a safer place. That's their mindset I get it.
Martin: What I heard him saying when he said that was, we're trying to build a human-machine team that will be the safest thing on the road, and that's our approach to this. Those are the kinds of things where I think we need a lot of focus. I think to your question, related to some of the questions that you had sent prior.
Martin: To understand how good these algorithms are, best practice is to identify a human performance measure. We need to have a measure to determine if the machine, if the AI is performing better than humans. If we don't have that, then we don't necessarily know how well the system is performing.
The Human-Machine Teaming Angle
Martin: That creates uncertainty, which leads to lack of trust in the system. As you think about this, I encourage you to go through that and say, okay, well, how would we measure? Because some of these things it's hard to measure human performance, but those are the ones that are easiest to automate.
Martin: Because then you know how well it's working. People who are familiar with the process can see it. And they feel good about what's going on, as opposed to having something happen behind a curtain pumping out a bunch of widgets. It all seems good until it's not.
Eric: You get that crash.
Eric: Not in a car but in something else. I get it. I do love the human-machine teaming angle. Arika your millennial friends, your gen Z, how do you think they'll handle that?
Arika: I think it makes a lot of sense. We believe that we can fully rely on technology, but what we've heard here today is just that. That there still has to be at least at this point, some human element to even how we look at AI or machine learning. I like the term human-machine team.
Eric: I actually think millennials will be more apt to adopt it because they're so much more used to tech in their world. I get a lot of pushback from customers. I've never seen artificial intelligence in the real world. I've never seen machine learning work well. There's this old school belief that humans can do it all or can do it better.
Identifying Best Practices
Eric: But there's not necessarily in cyber an acceptance that maybe driving too would be. There isn't an acceptance that the machines are very good at certain activities, like calling through tremendous amounts of data and searching on patterns or looking for patterns. People just aren't wired that way. Most people, I should say. Most aren't wired that way.
Arika: Bring more efficiencies as well. We shall see only time will tell.
Eric: Martin what do you think?
Martin: One of the things that we did at CISA, was to step back. This was about a year ago. And doing an analysis on what would be our criteria that we would put in place for determining whether or not we would automate something or not?
Martin: We wanted to make it really simple. We wanted to make it something that a program manager or somebody who's not necessarily well versed in artificial intelligence, but had a critical function.
Martin: Some guidelines that they could use to determine what would be a good task to automate and what would be a task to automate with great care. That was where we got to identifying some of these best practices. Things like this metric that we just talked about, human performance metrics.
Martin: But the things that we really focused in on were, you want to focus on automating high-impact, low regret, low complexity decisions. Unpacking that a little bit. You want to get the most bang for your buck. If it goes badly, you don't want to really regret it too much.
Choosing Places to Pilot AI for Cybersecurity
Eric: Low consequence. You don't want to crash the car and kill the occupants of the vehicle or somebody on the street.
Martin: Correct. Then back to this low complexity. That's really another way of saying something that's understandable. There's not a lot of uncertainty around that particular process. So that when folks are trying to determine how good they feel about the machine doing it, they can pretty much tell what's happening.
Martin: Those are the things that you want to start off with as you're choosing places to pilot artificial intelligence in your environment. So that you can build a good working knowledge of it. You can gain a lot of trust with the system and you can understand when things go wrong what it was and not have a big mess on your hands.
Eric: Let's go back to the C-3PO example I brought up. Incredibly intelligent and always precise in its data. You know the limitations of C-3PO right?
Eric: But what a human-machine team. I know it's a very basic Sci-Fi example, but almost using him to provide the data that the human just doesn't have in their head or can't do calculations that quickly. Low consequence though if you don't know the answer.
Eric: Arika, where do we go with this? Where do we go in a decade, two decades from now we're 55 years in, what do you think?
Arika: That's why I'm telling you guys to watch Black Mirror.
Eric: I feel so old. I feel like the Jetsons I just want to make things happen. I'll check out Black Mirror.
An Enterprise-Wide Conceptual Data Model
Arika: That's the type of things that they cover is everything, just from the way we work. There's a lot of episodes on actually security issues, things like that, but really taken from an AI machine learning perspective. They go about 20, 30 years into the future in most episodes, so check it out.
Eric: Martin what's next for us with AI? Where are we going?
Martin: I think as we talked about, Gartner identifies they've actually produced a lot of really good research on artificial intelligence. A prepare phase, which is where most organizations are. A lot of what I've talked about today are things that are in this prepare phase. It means you're preparing your data so that you can use it appropriately.
Martin: You're identifying criteria for determining which functions should be automated, as we just talked about. You're identifying pilot use cases. It says that what we've done is built this enterprise-wide conceptual data model. It's something that we use to identify all our data, how it interrelates, ensure that authorized use is maintained.
Martin: I really want to score that authorized use is front and center in our minds. That's easily accessible and it's prepared for AI tasks. Things such as labeling that you're going to need to do in order to use the data. We talked about identifying criteria when you're automating.
Martin: Then our cybersecurity use cases that we piloted our security orchestration automated response incident triage and security analytics like for the CDM program. So that we can rapidly analyze the data that we have and do something useful with it.
Humanitarian Assistance and Disaster Relief
Martin: One of our pilots which I'm involved in now is, we talked about I think, is enough being done, is a lot being done? Well, there's a lot being done in the government. DOD's joint artificial intelligence center has been big. They've been creating a lot of different kinds of capabilities. We're piloting one of their capabilities. It's a humanitarian assistance and disaster relief function.
Martin: It's imagery analysis that's being looked at as a termination. Whether or not it would make sense for us to use it for our response function under the Emergency Support Function 14, this is cross-sector coordination. This is part of the FEMA support for determining supply chain. Imagery analysis of US cities and determining whether or not, which is normally done for disasters.
Martin: To determine how far are floods going in, that kind of thing. Can we also use that imagery analysis to determine impacts to supply chain so that we get advanced knowledge or real-time knowledge of how supply chains could be impacted in this cross-sector support function under FEMA? There's a lot of things like that that we're looking at that hopefully will show some big benefits.
Growing Up in the 80s
Eric: Sounds good. Let's go as quickly as we can. I think we need it. Just now with COVID-19 and everything, it's a time where we need help. We need faster capabilities. That sounds awesome.
Martin: Certainly just go to a grocery store, we're seeing all the supply chain issues I think that maybe folks had, you were talking earlier about growing up in the 80s.
Martin: I grew up in the 80s and we certainly didn't have all these things on the shelves that we have today and we've come to really expect to enjoy them to be there and now when they're not there, it's surprising.
Eric: Now we did have toilet paper though.
Arika: Well, thank you Martin for joining us. I think this has been definitely a fascinating conversation, and it will be interesting to see what the future brings for sure.
About Our Guest
Martin Stanley, Senior Advisor for Artificial Intelligence, Cyber and Infrastructure Security Agency (DHS/CISA) leads the development of Artificial Intelligence strategy for the Department of Homeland Security Cyber and Infrastructure Security Agency.
He previously led the Cybersecurity Assurance Program at DHS and the Enterprise Cybersecurity Program at the U.S. Food and Drug Administration. Prior to his federal service Martin held executive leadership positions at Vonage and UUNET Technologies.