Why Do We Need Ethical Frameworks and Regulation for AI?
Forcepoint's 2024 Future Insights Post #4
0 minuti di lettura
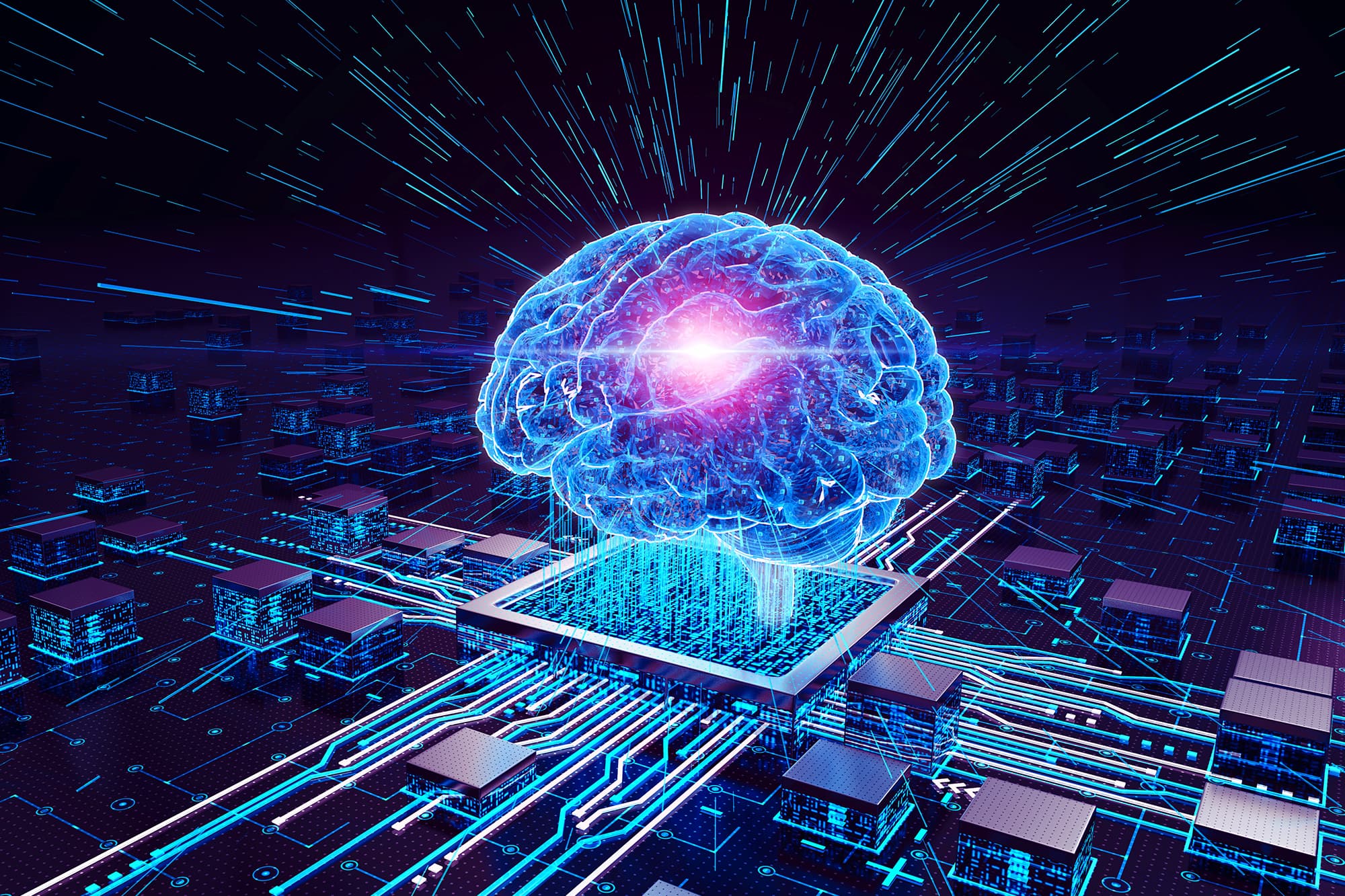
Nick Savvides
In recent years I’ve been asked by organizations to speak on the security ramifications of data poisoning, which has become a major concern for organizations working with artificial intelligence (AI) and machine learning (ML) systems. When you’re talking about the methods and motivations of attackers, data poisoning tends to present a relatively tidy and well-defined category (expertly covered in the prior post by Audra Simons).
But if you’re looking primarily at the impact on your systems, as is usually the case on cybersecurity teams, it can be tricky to immediately distinguish deliberate attacks from the unintentional effects of how AI systems are designed or where the data that they train on is sourced from. In this post, I’ll examine some systemic problems that afflict AI/ML systems, with the goal of explaining why ethical frameworks and regulatory governance are important to help AI function efficiently and equitably.
How can AI go wrong without attackers?
It’s possible to create an AI advisory system that trains on real and accurate data but ends up producing unethical outcomes. For a basic example, we can look at predictive text. Early ML models for predictive text were trained on public domain documents, typically digitized newspapers and books held by the US Library of Congress or other archival libraries like the National Archive in Australia. They would “read” the text and build models based on the recurrence of words in proximity to each other. The first predictive text libraries used in smartphones then inadvertently became somewhat sexist and racist. For example, writing the word “engineer” would cause the model to generate masculine-coded terms because the text of that era reflected the prevailing attitudes of the time. After the problem was identified, the models were tweaked to correct for this source of bias.
Another way that bias and unwanted elements can creep into AI models is through the crowdsourcing of datasets. A lot of the data that is used for training AI actually comes from human input, often through crowdsourcing platforms like Amazon Mechanical Turk. ImageNet is an example of a visual database that employed crowdsourcing to label its many images, leading to instances of racial bias (as well as some overtly racist language) that could then be absorbed by AI models. Whether training data is drawn from publicly available documents or from crowdsourcing, transparency is needed so that systemic problems hiding in datasets can be identified and mitigated.
To understand how serious the human impact of compromised advisory and automated decisioning systems can be, let’s look at criminal sentencing. Across the democratic countries with high levels of individual freedoms and accountability, there is a common theme of overworked lower courts (e.g., magistrate courts, trial courts, district courts), with judges and magistrates under extreme case loads. In such scenarios, a significant degree of operational efficiency can be gained by reducing the time it takes for sentencing. When a judge or magistrate needs to come up with a sentence after a verdict, they need to look at legislative obligations, precedent and community expectations along with the severity of the crime, and this takes time. In light of this, many jurisdictions have turned to AI to assist in looking at case information, running it through models and then providing sentencing recommendations.
Unfortunately, much like the language models, these systems are trained on old and long datasets and often make recommendations that are reflective of less enlightened times, recommending longer and harsher sentences for people of certain ethnicities and demographics. With the judge overworked, and the human tendency to believe the machine over everything else, this has resulted in judges applying the machines’ output over their own better judgement, leading to some rather racist and clearly disproportionate sentencing in a number of cases. Lack of transparency relating to how the AI models work and what data they train on has contributed to the problem.
There is one final problem to examine: the non-virtuous loop. This is where AI is used to generate an output which on its own is acceptable, as today’s many generative AI tools do; however, when this output is then used to train other AI models, it can cause the escalation and amplification of undesirable effects, resulting in outputs that are nonsensical at best and destructive at worst. In the case of the sentencing system that we looked at, should this go unchecked, future models will further discrimination and disproportionate sentencing. If an AI image generator gets trained over other AI-generated images, it can lead to downstream generation either looking all the same or nonsensical.
This type of AI model degeneration can be particularly severe in organizations training models over their own customer data, using models to generate synthetic data and then applying learning to the outputs. While the nonsensical can often be isolated quickly, more insidious are the inaccurate or deviant results that are hard to detect but have significant impact on downstream decision making or analysis. For example, a financial institution might model customer profitability using a set of models, which are then used by other models to generate synthetic customers, that are then used to generate models of how the institution’s profitability would change, or how specific customers are likely to perform over time. In such a case, customers could be denied access in a classic “computer says no” way without anyone knowing why the computer said no.
What does this have to do with cybersecurity?
When I talk about this subject, it’s at this point that people tend to stop me. “Okay, that’s terrible – but what’s it got to do with cybersecurity?” Unfortunately, it has *everything* to do with security.
We are increasingly dependent on AI for all parts of cybersecurity. It started with malware (moving from signatures to behavioral and feature analysis), then it was log analysis (moving from correlation to anomaly detection and user behavior analytics), now it’s everything. Machine learning models and AI decide if you should get access to a resource, if a user is presenting a heightened risk, if a resource is safe to access or if a malicious actor is inside your data and not just your systems. We cannot avoid AI, as it’s the only way we can scale our operations in the asymmetrical cyber battlefield. Every new piece of software or service will have an AI or ML element to it; in this sense, it will be similar to what the cloud was to software and applications 15 years ago. Applications have progressively moved into the cloud, and those that haven’t have had cloud principles applied in their private environments. In fact, in cyber we will move from using AI in defensive and detection techniques to deploying them in an adversarial manner.
But still, how do these issues affect cybersecurity directly? Again, tools used for cyber purposes are all susceptible to the dangers described above. Imagine a scenario, for example, in which you have trained an AI that looks at your data loss incidents and user behavior signals. The training data will be historical from your organization; did this data suffer from inadvertent poisoning from badly tuned policies? What happens when your AI is now locking legitimate users out of systems or denying access to resources because the training model ended up in a non-virtuous loop amplifying the importance of outliers? What happens in a scenario in which your AI incorrectly decides an employee is harassing someone or is at risk of self-harm?
What is there to do about it?
My goal here has been primarily to help you understand how unintentional bias and data poisoning occurs and how severe the human impact can be when these problems go unchecked. I’m interested in conveying why ethical frameworks and regulation are necessary for AI and not just a distraction for organizations as they pursue their bottom line. But I thought I’d end by briefly pointing in the direction of what is being done in this area.
Ethical frameworks
Establishing best practices for ethics in AI is a challenge because of how quickly the technology is developing, but a number of public- and private-sector organizations have taken it upon themselves to deploy frameworks and information hubs for ethical questions. Here is a small sampling of what’s out there:
Regulatory governance
While work on ethical frameworks may come across as a bit haphazard, actual regulation of AI is truly in its infancy. The EU AI Act is one of the first major pieces of legislation to establish regulatory governance of AI applications. Now in the US, President Biden has just issued an Executive Order to establish standards and guidelines for both the development and usage of AI. This is the broadest set of rules in the US, building on some of the laws US states have passed on AI usage, and is worthy of analysis and study in its own right.
Furthermore, the World Health Organization has proposed regulatory principles relating specifically to healthcare concerns. Of course, this is to say nothing of how existing data security and data privacy regulations such as the GDPR impact artificial intelligence usage.
The future is regulated
All of this activity is likely to spark increasing amounts of regulation in the major economies and trading blocks which for a while which could lead to an increasingly piecemeal regulatory landscape at least for now.
I think it’s safe to predict that the current “Wild West” era of AI and ML will fade quickly, leaving organizations with a sizable compliance burden when they want to take advantage of the technology.
Dealing with all of this will be difficult, but I hope I’ve successfully demonstrated that approaching AI from the perspective of ethical design and regulatory compliance is critical if we want to protect the many people, users and otherwise, who are impacted by these systems.
Nick Savvides
Leggi più articoli di Nick SavvidesNick Savvides serves as Field CTO & Head of Strategic Business, APAC at Forcepoint. In this role, he is responsible for growing the company’s strategic business with its key customers in the region. This involves taking the lead to solve customers’ most complex security issues while accelerating the adoption of human-centric security systems to support their business growth and digital transformation. In addition, Savvides is responsible for providing thought leadership and over-the-horizon guidance to CISOs, industry and analysts.
Nell'articolo
X-Labs
Get insight, analysis & news straight to your inbox
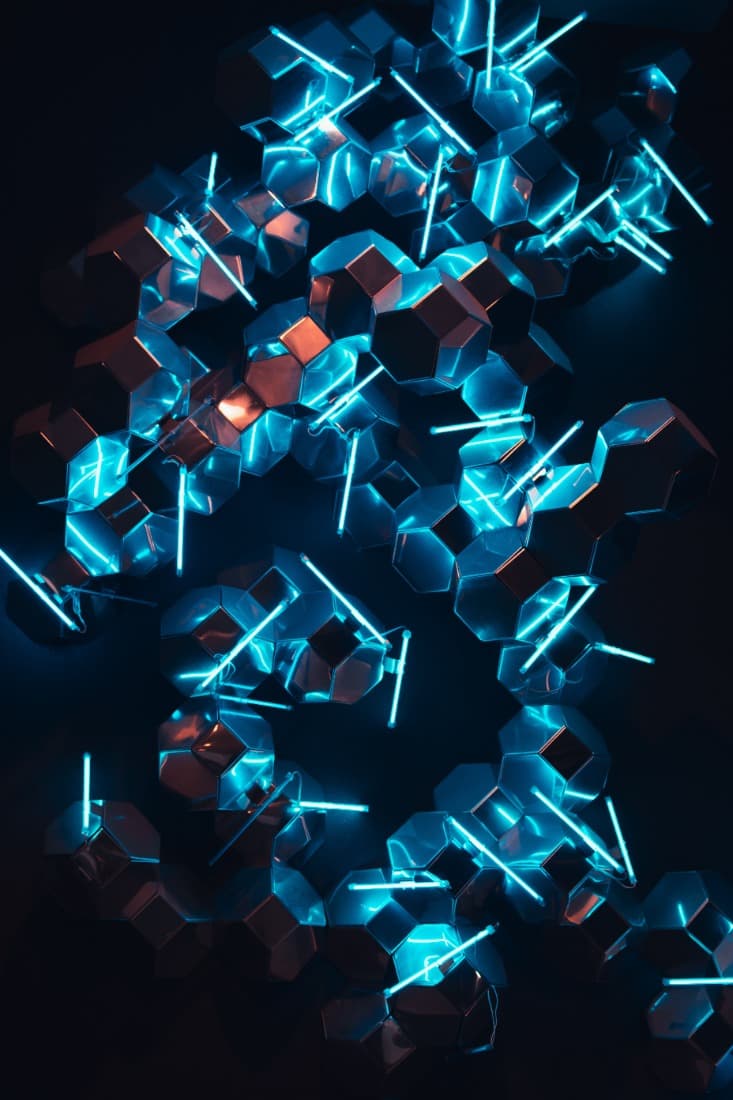
Al Punto
Sicurezza Informatica
Un podcast che copre le ultime tendenze e argomenti nel mondo della sicurezza informatica
Ascolta Ora