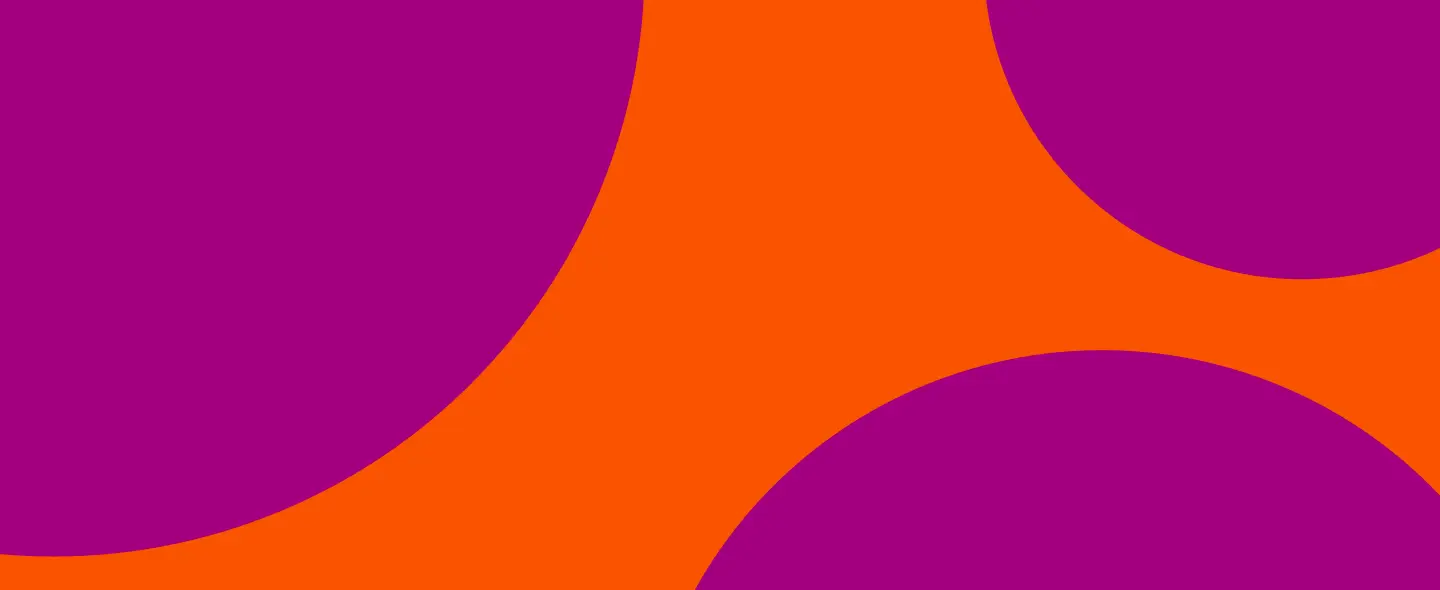
REPLAY: Data Governance in Today's Data-Driven Culture
Share
Podcast
About This Episode
In this week’s replay episode, we welcome to the podcast Lauren Maffeo, Senior Service Designer at Steampunk and Author of "Designing Data Governance from the Ground Up." We double click into data governance – particularly in the age of AI – why it matters, the risks of not having a governance framework, the opportunity AI governance can deliver organizations, as well as best practices when developing AI governance.
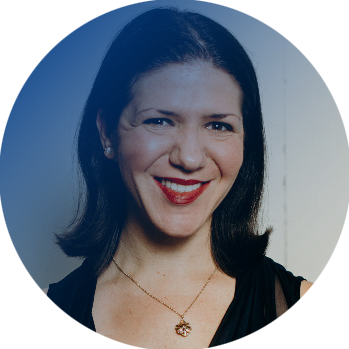
We also wade into the emerging hot topic of data poisoning, the risks it poses to AI models and behaviors that lead to anti-patterns. She also shares inspiration for her book and the path to bringing that to market! Learn more about Lauren’s book, Designing Data Governance from the Ground Up: Six Steps to Building a Data-Driven Culture.
Podcast
Popular Episodes
Podcast
REPLAY: Data Governance in Today's Data-Driven Culture
[0:59] The Crucial Role of Data Governance in AI Deployment
Rachael: I'm so excited for today's guest. We've got Lauren Maffeo. She is a senior service designer at Steampunk. She's also an author of Designing Data Governments from the Ground Up, which was adapted to a LinkedIn learning course, which is kind of amazing. I've never met anybody who did that. And then on top of all her other accolades, she's also an adjunct lecturer at George Washington University. Lauren, welcome.
Lauren: Hi, Rachel and Audra, thank you so much for having me. I'm excited to be here.
Audra: Brilliant to have you. So shall we jump straight in? We have one of our favorite subjects beyond cybersecurity is talking about artificial intelligence and the impact that it's having just across the board. And so I wanted to hear your opinion on how the rise of AI impacted data governance and how organizations manage their data.
Lauren: I love this question because Iif I'm being honest, kind of been slapping myself this year thinking if I had just named my book Designing AI Governance from the Ground Up, I might have maybe five times the sales now because in all seriousness.
The biggest thing, I really try to hit the point home is that in order to succeed at deploying accurate, successful, trustworthy, and transparent AI, you have to have data governance. It is not a nice to have. It is a must-have. I still think even in our industry. There is too big of a gap between acknowledging the role that data plays within AI, which is that it's the foundation of any AI that you deploy.
Navigating the AI Landscape
Lauren: I mean, at the end of the day, AI is data and it's using technology to find patterns within often large amounts of data. And that disconnect is something that I see the gap decreasing. But I also think we're a little bit far from where we need to be.
I think there's more acknowledgment in the industry that data governance writ large is not where it is supposed to be in order to deploy successful AI. And I would argue that that drives the over-reliance on large language models like ChatGPT. Because organizations know that they are not prepared to deploy their own proprietary AI with the data that they have and the lack of governance.
And so then they rely on external models, which may or may not be validated, which may not be updated regularly, which might have data drift. And so I think we are bridging that gap between data governance and AI. But I still see a big rush to the AI side of things without acknowledging the backbone. And I would say that that's true in the media as well. I mean, I read quite a lot of tech news.
I read the Wall Street Journal every morning, which covers AI ad nauseam. And I don't see data governance entering the conversation as much as I think it should. I think that's going to change. I think if we talk in five years. We'll be having a different conversation similar to cybersecurity and how five years ago nobody wanted to talk about cybersecurity. Especially in open-source communities. They basically would stick their fingers in their ears anytime you brought up security issues with open source.
Anticipating the Data Revolution
Lauren: And now five years later, there have been many attacks and many developers have had to work more than one holiday to try to patch issues. And I think we're going to have something similar happen with data. Humans are inherently reactive people. And I do think it's going to take something pretty big to get folks to acknowledge the role that data governance plays in order to drive that more high-level substantial change.
Audra: I think that the problem is it's not just something really big. It always has to be something bad to happen. A hundred percent of people take it seriously. And unfortunately, having read some of, well, quite a few different articles around this. There's that whole when it comes to AI, if you feed it with garbage, you will get garbage back out. So can you talk around what are some of the risks associated with not having an AI governance framework in place?
Lauren: Absolutely. I think the biggest point on this note that I'd love to hit home is that there's a big discussion about bias in AI. And how the data that is used to train large AI algorithms, which affect large numbers of users are not diverse enough or transparent enough. And part of the reason I was interested in this book is the fact that I really believe that data governance is a form of AI ethics.
You can't have one without the other. The biggest benefit of data governance is creating more transparent, trustworthy AI. There is academic research showing that people have more trust in algorithms when they can see how the algorithms were trained.
The Role of Data Governance in Navigating Global Privacy Landscapes
Lauren: Even if their output is often incorrect or less accurate. And so that transparency aspect is going to be really crucial over the next few years. I know Audra, you're in the UK, and GDPR of course is the law of the land there.
That means that European users and UK users have a lot of rights to their personal data. They have the ability to ask organizations how the data that they've collected on these users is being utilized for financial gain by those companies. And if organizations are not able to give that information, then they are liable to lose I think up to five to 6% of their annual revenue.
Now, if you have a large organization like Google, Facebook, or Microsoft, we all know that they have the money to pay those fines. And that's part of the issue with, I would say that's an antitrust issue with data. Because those types of companies that own the biggest data sets are too big to fail. Because again, they can effectively just break the law and then pay the fines. But for the average organization, losing five to 6% of your annual revenue in this economy is enough for your business. So it's something that leaders do have to take seriously.
And in the US we do have, as US citizens far fewer rights to our personal data. Especially at the federal level. But we are seeing legislation at the state level, which is giving users more rights and autonomy over their data. California is the biggest example, but we are also seeing legislation in Virginia and New York.
[8:06] Islands of Governance
Lauren: And so I think that in order to create products and create AI, which is not just accurate but also more transparent which is going to be essential moving forward. Because users are going to have more rights over their personal data. And if organizations not only want to use AI but stay in business doing so. Data governance is going to be essential.
Audra: So I was reading the state of Hawaii is looking to create a statewide data governance framework. What does that even mean? So statewide, this is how we deal with data. I mean, can you talk about that at all?
Lauren: I can. So without knowing the state of Hawaii, it's hard to be incredibly specific. But one thing that comes to mind is that when you think of Hawaii as a state, this is actually a great analogy for federated data governance. That's probably only a conversation I can have on a podcast like this. Because most people would not think of federated data governance when they think of Hawaii. But if you think about the respective islands of Hawaii, that's a great analogy for a federated approach to data governance.
And basically what that means is that it's a concept that pairs subject matter experts with technical talent to create the standards for data quality and consistency in their respective data domains. And then what happens is those subject matter experts who create the standards for governance pair with technical talent like data engineers. They operationalize those data standards for quality in the production environment and in the technical tools. Whether they're using Collibra or Informatica, which are pretty standard industry data governance tools.
Crafting a Strategic Data Governance Framework
Lauren: The idea here is that you have subject matter experts per data domain. So in this case, it would be if every island in Hawaii is its own data domain. You would have a subject matter expert per domain. And that's the person who leads that area. So they might manage a team. They also are the person closest to the data per domain. And so they're the best advice to write data definitions, to speak to data quality, to add and update data definitions in their data catalog.
Then what they do is they work with data engineers who can take the standards created by each domain subject matter expert. And put them into production. This step is really crucial with data governance. One of the biggest issues I see is that organizations. If they do have a data governance plan. It lives in a 60-page Word document that was last updated in 2005 and nobody even knows where it is.
So then as you can imagine, it never touches the tech side, the production environment. As a result, the technical talent really views the data governance as an out-of-date compliance document, which hinders innovation. If done that way, they're not incorrect because, in that state the data governance document does not dictate anything meaningful about the organizational approach to data. So when we think about Hawaii,
I think the biggest takeaway is considering that federated model where you have those subject matter experts per data domain, which in this case could be each island. Then you have that person paired with a technical lead to implement the data standards into one singular environment. That's another thing that I think is really crucial with any data governance framework.
Future-Proofing Data Governance
Lauren: You have to be very intentional about how you set up and design the technical environment. One of the biggest issues I see with data governance is that the data documentation and the data sets themselves do not live in a centralized place. So then that makes it very difficult. So if you have that framework that is prioritizing data domains and subject matter expertise per domain then I think you can start to make progress in the technical environment and really start implementing your data governance standards into the tech.
Audra: How do you keep up with it and keep it relevant though? Because one of the things about as soon as you've kind of trained your model, the model's out of date because the data changes, and things move on, how do you keep it relevant?
Lauren: That's a great question and I love that you asked this. This is one of the biggest differences when we talk about deploying data to production versus deploying code. Of course, you're going to have to update the code after deployment in many cases. But when you're working with AI, I think a lot of people just focus on getting to production, getting out of the dev environment. But then the reality is that's only the beginning.
Because as you say, especially if you're using AI-like machine learning. Machine learning relies on the algorithms constantly ingesting new data. We hope to improve the results of that algorithm. But if not, if left ungoverned, the opposite can happen. The quality of that model can degrade over time in what's known as data drift.
The Crucial Role of Data Governance in Monitoring and Mitigating AI Data Challenges
Lauren: Again, that's why data governance standards and data quality assessments are so crucial. Because the lack of them leaves teams completely unprepared to assess data drift to automate it. To get that high-level picture of which data points are being used in which algorithms.
So it's really important to set up data monitoring in your production environment so that you are able to spot issues as they occur. Because that's also essential for data transparency and data inaccuracy. Part of what makes training AI so difficult is that if you spot an error, you have to then roll back the model to the point at which you spotted the bias and address it at the source. So if you haven't set up data monitoring, you don't know the source of the error.
Then it becomes a goose chase to try to find it. So data monitoring is absolutely essential. You do have to set up data drift, and that can be as this is not a simple exercise that said. I think this really emphasizes why you at a bare minimum need conditions for past data that passes versus data that fails.
I've spoken to QA engineers at data conferences this year who say we have no data quality standards. As a result, I feel like I can barely do QA because I don't have the pass-fail conditions. So if you're looking for a place to start with data quality that is it right there starting with the very basics of pass-fail conditions. Because then the other thing to keep in mind is that the data used in your algorithms needs examples of both positive and negative classes.
[15:42]The Vital Role of Data Governance in Safeguarding Against Data Drift
Lauren: It needs to know what success looks like for data matches. It also needs to know what the data is not. So pass-fail conditions and defining those. Then operationalizing those standards in your architecture is really crucial to preventing data drift.
Audra: And beyond data drift, there are other more dangerous things can happen on purpose like data poisoning where you're actually poisoning the dataset or confusing the data sets to cause that drift intentionally. Have you come across that in your work in terms of intentional data poisoning?
Lauren: I would argue that to date data poisoning is not a mainstream subject. Or at least not as mainstream as it could or should be. But the reality is that as the volume of data grows and continues to grow exponentially. I think we're going to see more high-profile instances of data poisoning.
Data poisoning at its most basic level is a malicious attack that tries to manipulate the data in a training set for an AI algorithm.
So this is a case where a hacker would be intentionally trying to manipulate the data that goes into a dataset to produce a specific often negative result. This is a risk with open source data and algorithms that when it is open to anyone and everyone then in theory anybody could infiltrate it. So I think this data poisoning concept is something that emphasizes why the chief data officer really needs to be working hand in hand with the CISO. Because data governance has a very essential cybersecurity component to it.
Safeguarding Data Integrity
Lauren: And you have to be very clear also about what personally identifiable information looks like for your business. Because that's the sort of stuff where if there's a data poisoning attack on social security numbers on highly sensitive data that could cause catastrophic results. So data poisoning is certainly something that I think needs to be assessed as part of any data governance framework. My book talks about Gartner's seven-step framework for data governance and emphasizes that you can't have one without the other six.
And cybersecurity is of course a crucial component to it. I think preventing data poisoning is the big task ahead of any CDO and CISO in 2024. But I also think there's a lot of opportunity. We know, for instance, that employees are the most likely source of a cybersecurity slash data breach at organizations. I do think that we've seen CISOs step up to the plate in terms of creating organizations that are more aware of what phishing attempts look like.
How to enable factor authentication of how to create micro-learning courses, which teach employees how to spot malicious attacks. This is something that I think Steampunk does exceptionally well. Teaching all of us to be on guard for hacking attempts in any high-profile/highly regulated industry. Again, not a nice to have. It is a must-have. And I think that CDOs can learn a lot from CISOs who have taken the lead in creating more cyber-aware workforces.
Detecting and Mitigating Data Poisoning in the Era of Advanced Data Governance
Audra: I think one of the biggest challenges that their leaders will face is identifying when data poisoning actually happened. I think that's one of the biggest challenges that they'll hit. Because Google had it for a period of time where they weren't able to identify spam. Because their datasets had been infiltrated and poisoned so that things previously that were like spam were not spam. So lots of people spammers out there were having an awesome time kind of just like, oh, it just passes through. It's not spam because of that. And it took quite a period of time to identify that it happened.
Lauren: Yes, I agree. And I think that's going to become more common. I think anytime you use a platform like Gmail there is a very large data set that could be infiltrated. Those I think are the most high-risk opportunities for data poisoning because you can do it at scale. This is an interesting twist on data governance data. Because I often say when I'm talking about bias in AI, it is very often not intentional. It isn't so much that data teams and data scientists set out to create algorithms that are biased for or against certain types of users.
It's that there's indirect bias in the data sets where sensitive attributes like race or religion correlate with nonsensitive attributes. They often do this in ways that we cannot see due to black-box algorithms. So then that's how you get products that are biased towards some users or work to the detriment of others. However, data poisoning is quite different because this is very much a malicious attack on AI and data sets.
Data Governance in the Crosshairs
Lauren: I worry if I'm being frank about the future of warfare because I mean many of us in the industry talk about how future wars will increasingly be fought online as opposed to in person. So I think in terms of national security, data poisoning is huge. And I think that's something that absolutely, again, it's not a nice to have, it's a must-have.
When we think about what that looks like, I see data poisoning being a huge risk for warfare in the future. We're speaking at the end of 2023 ahead of a year in which many global elections will occur in global democracies. I do see data poisoning as a real risk there. I hope I'm wrong, but it's certainly something that I think is under-discussed in the industry to date.
Rachael: Absolutely. I don't even trust anything anymore, Lauren. I mean, I'm a big fan of TikTok. I'm on the older side of the TikTok audience. But I literally will go I'll see something and then I go check it against 3, 4, 5 sources to see if is this even true. Because the videos are very compelling, deep fake videos, misinformation.
And I put something in the chat GPT and see what I get back, and I still have to go double, triple check that as well. It's a crazy time. You don't do all that work to go verify that something is true. And even sometimes after five sources, Lauren, I'm not sure that I've actually kind of come to a conclusion one way or the other. And that's been troubling me lately.
[23:10] Building Trust in the Digital Age
Lauren: Well, I'll be honest, I'm impressed that you, quintuple-check your sources because the reality is that many of us don't do that. We don't take the time to validate our source. And one of the biggest things, and that is not a good thing. It's certainly not a good thing to be paranoid. Having said that, I am impressed that you even took the time to validate the source of this information.
Because I think that's another risk of malicious attacks like data poisoning and deep fakes. I mean, we could have a whole episode just on deep fake creation and what that looks like because that's another big concern of mine. Especially ahead of 2024, is how it is getting more easy to create fake content.
The audio is huge, and that's going to become even riskier. So I think the biggest thing I worry about is that ultimately the goal of attacks like that is to make people question reality and not trust anything. And I do think that's an enormous risk that I do see that is acknowledged amongst people in the data and computer science and media literacy spaces. This concept that it is so crucial for people to have clear, consistent trustworthy sources.
I am increasingly, as a former journalist, convinced that the demise of locally produced news is the root cause of a lot of this. Because if you look at the literal death of community reporting across the United States. I imagine across parts of Europe, if not all of Europe, that does create truly a destabilizing effect. Because what's happening is that we all collectively move more online. We lose those people in the community who are entrusted to report accurately and consistently on that community.
Unveiling Data Governance Anti-Patterns and Fostering a Culture of Maturity
Lauren: And so when we lose that local touch, then it does ultimately lead to a world where there is too much information for us to consider and internalize at scale. And that causes us to just question the essence of reality. So the biggest takeaway I can give to people is to find a local source of news hopefully you do have one wherever your audience members live. But please financially support your local sources of journalism because that is a huge culprit for a lot of the issues we're seeing with misinformation and the lack of media literacy today.
Audra: So can I bring one other risk area in terms of anti-patterns? Can you talk about anti-patterns in data governance and what kind of common behaviors lead to anti-patterns?
Lauren: Sure. So anti-patterns I think of in data governance as being the poor practices that relate to data governance. So any patterns are performed at scale and they have a detrimental effect on your data governance. The biggest issue I see, the biggest anti-pattern I see with data governance is the lack of it. You would be pretty stunned to see the lack of data maturity that most organizations have. And this is not limited to one industry, to one country, to either nonprofits or to corporations.
I was a research analyst at Gartner in the States for several years before joining Steampunk. I reported on trends in the cloud business intelligence space, which is where I started researching various AI techniques and how they could be used to solve specific business problems. And for years now. Gartner has been reporting on not just the possible trends that organizations could take advantage of, but also the lack of data maturity in organizations of all sizes.
Data Governance Awakening
Lauren: The reality is that seven to eight years after I started doing that initial work, we have more data produced and ingested than ever before. And if anything, the data maturity of most organizations is going down. So the lack of data governance, the lack of standards, the lack of quality assessments and what those look like, the lack of cross-collaboration across silos. Those are all anti-patterns as far as I'm concerned.
Again, we as a society so far behind where we should be when it comes to data governance. And that does, again, create over-reliance on third-party large language models like ChatGPT, because we have not done the inherent retrospective work to get our own models where they need to be. I do see that changing out of necessity over the next five to 10 years, but right now, those are the biggest anti-patterns that I see when it comes to data governance.
Audra: But do you believe that whether it's governments or enterprises actually understand their data?
Lauren: No, I don't. And I think that's also the biggest thing. That's one of the biggest problems I see as well is that I constantly talk to clients and to senior leaders at organizations who say we have a two-pronged problem. We don't have enough technical talent on the data side to facilitate all of the requests that we get. We also do not have a high-level strategy for data governance. So as a result, we get all of these ad hoc requests, which are classified as urgent, whether they are or not, and we are expected to respond right away almost like a queue. And so that's another issue I see is this highly reactive environment.
Strategic Foundations
Lauren: We see this too with AI investments once chat GPT exploded in mainstream popularity earlier this year. I mean, think about how many you read in 2023 about investing in AI, and then nothing happens and organizations wonder why they wasted in many cases, millions of dollars on these investments that didn't pan out.
It really does come back to the lack of strategy and the lack of processes and standards. And I know that that can sound very broad. The reason it's broad is because those are going to be different depending on each organization because one organization's data governance strategy is going to differ from another's, but you still have to figure out what data governance looks like in your organization. What is your plan to manage the data that you have at scale?
That's essential. I just read an article about an entrepreneur who said that she started taking the entire month off of December to exclusively focus on strategy planning for the forthcoming year. And so she does not take meetings with clients during December. She does not respond to requests. She really uses the month of December to plan ahead for the forthcoming year. I know that sounds extreme and that the reality is most organizations can't afford to do that, especially if they are public, that is something that might not be able to happen to the same degree.
Having said that, she was using this practice as an example of a strategy that has transformed her business. And so I do think that the lack of focus on strategic planning is what becomes an issue. And I again think it's really essential for data leaders to do that introspective work of even figuring out where their data lives.
[31:35] Unveiling the Data Labyrinth
Lauren: I can't count the number of clients I've worked with where I come in as part of a team to design a data architecture environment to figure out what data mesh is going to look like to help clients implement that federated approach we talked about. And so one of the first questions is always, oh, where does your data live? And they're like, I don't know, dispersed. I mean, they literally don't even know which server this data set lives on. They have a mix of on-prem and cloud.
By the way, those on-prem cloud tools are often not integrated with each other. So then that creates problems. They often have the same dataset living not only in different environments but sometimes the data in the same dataset is different. And so I don't think that most organizations today have enough of a handle on their data, and that's why the introspective work of being honest about where you're at is really crucial. I think most leaders know that they are not prepared to meet the moment when it comes to their data, and so they push it aside. And again, that is something that can't continue moving forward.
Audra: So can I ask, what was the catalyst that inspired your writing, the book you wrote, designing data governance from the ground up? The way you talk about this and your passion, was there a catalyst or was it just a mountain of the many reasons that you needed to put it to paper?
Breaking Chains
Lauren: That's a great question. I do remember the catalyst being that I was working with a particular client whose job it was to disseminate data for public use. And when I conducted my user research and finished the discovery phase of the project and I got a handle on their processes for disseminating that data to the public, I realized that there was no automation involved. Disseminating this data was a process that took days to complete.
Several people were involved in very manual ways and there, so the inefficiencies were just astronomical. And I realized that if an organization that existed to share data with the public was operating this way, then this was truly a bigger problem than I even realized. So that was the catalyst for me pitching and ultimately writing this book was confirming just how widespread the problem is and then doing what I could to propose a different approach to it.
So as a surface designer, I always say that I'm the user advocate on projects. My job is to figure out who the core users of any product or service are and to speak with them using qualitative conversations to figure out what their experience is with that product or service, what they like or dislike about it, and where they encounter friction. And then ultimately, my job is to not only capture the current real state of that user experience but to identify areas of friction, which I can then work with my team to create solutions on. I don't see most organizations thinking of data governance or data science as a design challenge or the approach to solving it as a design challenge, but I wrote this book to propose a different way of viewing this problem.
Navigating Cultural Shifts in Data Governance
Lauren: And so I hope it has made an impact and will continue to do so because the more I do this work, the more convinced I become that this is not a technical question to solve. This is really a cultural transformation. And designing a data-driven culture is not something that happens by accident. It is a strategic approach. It's a strategic choice that organizations make. And I hope that I can move the needle in convincing data leaders to take that approach.
Audra: I think the way you lay it out in the framework and provide steps that they can take exactly, it gives a really good concrete approach. We have so much data and it's like what's important, what's not and how do we use it and how do we benefit our business from it? How do we benefit our customers from it? All those sorts of things. Having a practical approach is incredibly useful. And your book is in my Amazon account, so on its way here.
Lauren: Oh, fantastic.
Rachael: And we'll link to it in the show notes as well, Lauren. We'll definitely let our listeners get the link as well.
Lauren: That would be fantastic. Thank you so much.
Audra: And I know we have time. I'd really like it if you could provide us, Lauren, I love origin stories and you've had quite a varied background. I find these stories wonderfully inspiring, so if you're happy to share your origin story with us.
From the Newsroom to Data Governance
Lauren: I would love that. So part of my impassioned plea to support local news is personal because I thought through university and even graduate school in London that what was going to become a journalist of some sort. I chose to attend college at a university in a large city on the East Coast in part so that I could have easy access to journalism, internships, and newsrooms. I did live-on-air radio reporting for an FM station in Washington DC I interned at New York One, which is a very prominent cable news station in New York City. It sounds like it, Rachel.
Rachael: I lived in New York for 15 years and I'm a big fan of the New York One.
Lauren: Yes. If you've lived in New York City for any period of time, New York One, and I mean, that was my favorite summer definitely of college, if not ever. That was such an awesome opportunity. And I did pursue freelance news and digital news reporting for the first year out of graduate school. I loved the work as much as I thought I would.
And that was very fulfilling. But what I realized pretty quickly was that the industry was too precarious to support a full-time career. So I had to have a pretty honest conversation with myself about whether this career path was viable in terms of fulfilling basic adult needs, like saving for a 401k, maybe having a family, or having health insurance. I mean, these were fundamental questions that I had to ask myself. So after doing freelance reporting for a year, I decided to pursue employment in a different sector, the news.
[38:51] Connecting Tech Trends
Lauren: But the link between my career in tech and my career news is that I started my career reporting on trends in the tech and entrepreneurial space in Europe from London. And so I started my career in reporting as a tech reporter. At the time, I didn't care which sector I covered. I just wanted the clips that would help me land jobs. But I realized in doing so that I actually was very interested in tech, specifically AI.
As AI grew in prominence within the last decade. And so I transitioned to reporting from reporting about tech to working in tech. I started in marketing several years ago and then eventually transitioned to being a research analyst. And then finally, I'm currently a service designer and author. And so that's in a nutshell, my origin story. I think the common link between all of it is this genuine interest in understanding human behavior and how people interact with and are affected by tech.
And that is the big question that drives a lot of this work. I think too many people view data governance as an activity that happens in isolation with no real-world effects. I know from my own career how untrue this is. A big part of my work involves helping people see the link between the lack of data governance and the very real-world human consequences. Anything I can do to bridge that gap is what I feel is my life's work.
Audra: That's awesome. Fantastic. Thank you, Lauren.
Navigating News Reporting to Data Governance
Rachael: Absolutely. I love that pathway too. Some tech is such a great world too. I've been in tech for decades, but I feel like I learn something new every day. And I don't know if other industries are like that. But I just can't imagine working in any other world where things weren't changing seemingly daily. Right. And it's exciting.
Lauren: Yes. And Rachel, you actually just hit on another key reason why I wanted to work in news and work in tech. Now, one of the things I love about interning and working in the news is that you do something different every day. Even if the mechanics of your job are the same, you're writing new articles, talking to new sources, thinking about new ways to tell and frame a story, and tech is the same.
There's literally every day something new to learn. So if you're the type of person who likes learning, I think tech is the perfect career path for you. And I hope I haven't come across in this conversation as a pessimist when it comes to tech. The truth is that I also am a big proponent of data and tech and AI, and I'm very interested in Slash and excited by the opportunities that it brings.
Navigating Data Governance in the Tech Realm
Lauren: I think my frustration comes from not seeing that opportunity realized and from seeing most people take an approach to data, that involves burying their heads in the sand as opposed to thinking, how can we use this data that we have to build something really exciting for our users? I think there's a ton of untapped opportunity, and that data governance is a core part of getting you from point A to point B. So I also am a big fan of tech. That's why I work in the sector, and I hope that there is work forthcoming that will help more organizations use tech specifically AI more effectively and help realize their full potential with it.
Rachael: Absolutely. What an exciting path ahead. I think there's so much opportunity and you just have to harness it and take that first step, right? And it can be a game-changer.
Lauren: I completely agree.
Rachael: Well, I am sad we are at our time this week. Audra. Lauren, thank you so much for joining us. This has been such a wonderful conversation.
Navigating Tech's Transformative Realm
Lauren: Yes, it really has. Thank you so much again for having me. If folks want to find the book, it's called Designing Data Governance from the Ground Up. You can order it from any major retailer, and also you can order through your local bookstores. So I would be very grateful to hear from many readers and listeners who purchased the book and to hear their thoughts. And I hope that this was the first of many conversations that I'll have with you.
Rachael: Excellent. Love to have your feedback. Yes, don't worry.
Lauren: Please do. In all seriousness, please do, because I mentioned to you both before we started recording that version two of this book is certainly not out of the question. There are no plans at the moment to do that, but it's certainly something that's on my mind. I think right now before I would write another book. I would want to make updates to this one because again, the landscape is constantly changing. There's always more to unpack and address, and I'm a big part of when and if I will write version two depending on reader feedback and what they'd like to learn more about. So
Audra, please do reach out with any feedback and I hope your listeners will do the same.
Rachael: I will. Well, to all of our listeners out there, thanks again for joining us for another amazing discussion. And as always, don't forget to smash that subscription button.
About Our Guest
Lauren Maffeo is an award-winning analyst and designer whose practice includes writing and executing research plans, leading user interviews, hosting usability testing, and creating assets like personas, process and journey maps, service blueprints, and content strategy/migration. Today, Lauren works as a service designer at Steampunk, a human-centered design firm serving the federal government. Her first book, "Designing Data Governance from the Ground Up", is available from the Pragmatic Bookshelf.
Lauren is also a founding editor of Springer’s AI and Ethics journal and a former area editor for Data and Policy, an open-access journal with Cambridge University Press. She has presented her research on bias in AI at venues including Princeton and Columbia Universities, Google DevFest DC, and Twitter’s San Francisco headquarters.
Prior to joining Steampunk, Lauren was an associate principal analyst at Gartner, where she covered the impact of emerging tech like AI and blockchain on small business owners. Lauren has written for Harvard Data Science Review, Financial Times, and The Guardian, among other publications. She has also peer-reviewed technology research and books published by the GovLab at NYU, O’Reilly Media, and The Atlantic Council.
Lauren is a fellow of the Royal Society of Arts, a member of the Association for Computing Machinery’s Distinguished Speakers Program, and a member of the International Academy of Digital Arts and Sciences, where she helps judge the Webby Awards.