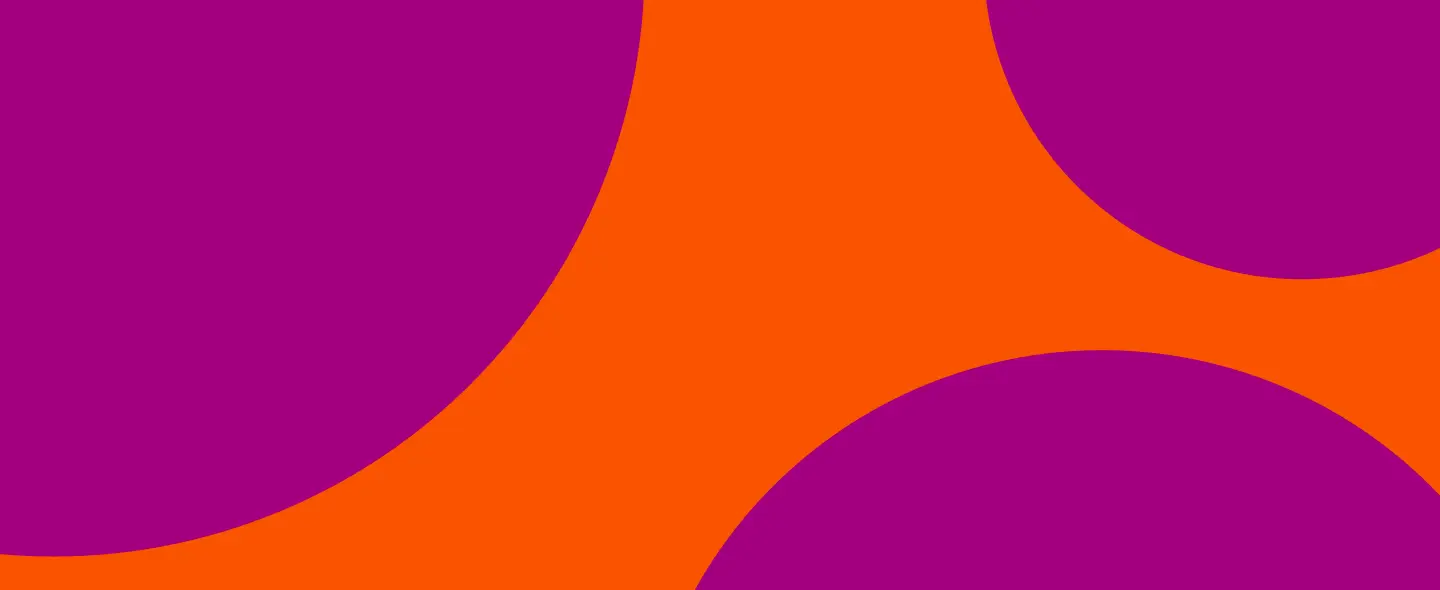
Replay: Human vs. Super Suit: Exploring the AI-Human Relationship
Share
Podcast
About This Episode
For this week’s episode, we replay a talk with Casey Ellis, Founder and CTO of Bugcrowd and Co-Founder of The disclose.io Project, to explore the risks and rewards of exploring AI technology, including concerns around the ubiquitous ChatGPT chatbot.
As the global race to AI supremacy intensifies, Casey shares his thoughts on AI in the workplace, as a cyber defense, and the future of regulation and the ethics around determining AI liability,
Podcast
Popular Episodes
Podcast
Replay: Human vs. Super Suit: Exploring the AI-Human Relationship

[00:44] Casey John Ellis on Cybersecurity Defense
Petko: I'm joined today by Casey Ellis, the Chairman, Founder, and CTO of Bugcrowd. He's also the Co-Founder of the disclose.io project. Welcome, Casey.
Casey: Thanks for having me.
Petko: You've had a long career, doing this for over 20 years as most of the folks, as our listeners are listening to. You've pioneered the concept of bug sourcing as a security service, right? Can you tell us more about that program and what you've been up to since then?
Casey: Appreciate that. I think a lot of people of my age and stage in this industry, I grew up fascinated by it as a kid and tripped over into it out of high school and everything went from there. So, from what I've heard, that's a fairly common story and some of the listeners might actually relate to that. With respect to Bugcrowd, what we did, we didn't actually invent vulnerability disclosure or bug bounty programs.
That was prior art, for sure. One of the earliest examples of a bug bounty program goes back to 1995. I think, with Netscape.
There's examples prior to that as well. But what we did was to pretty much pioneer this idea of building a platform that sits in between the latent potential and all the creativity of white hat hackers and security researchers that are out there, and then all of the different problems that we need to solve as cybersecurity defenders. My fundamental point of view is that cybersecurity itself is an intrinsically human problem.
We've just sped it up. Humans are perpetually a part of the solution when it comes to outsmarting bad guys as we go forward.
[02:20] Exploring AI: The ChatGPT Chatbot
Casey: The question I had in my head before I started Bugcrowd was, how do we scale that? How do we deal with the growing internet? How to keep my buddies out of jail on the hacker side, the folks that think bad but do good? Making sure that people actually understand that they're part of the solution, not part of the problem, all those different things.
So it's been about 11 years now and so far, so good. I haven't gotten tired of it yet.
Petko: Awesome. I mean, I'm glad that you're helping companies develop secure products and partnering the researchers with the companies. I think that's a great use case. There's something that's been really hot in the media recently and I'd love to get your thoughts on it. And if I throw it out there, I'm sure everyone know what it is immediately.
ChatGPT, I've been playing with it and one thing I've found is it makes the access to the ideas a lot easier.
If I wanted to attack a piece of software, it won't tell me how to do it. But I can ask very specific questions then it might help me find ways to attack it. I think it's that ChatGPT is going to open that up to so many others. Finding more vulnerabilities, maybe even potentially on the offensive. What do you think about ChatGPT? How does that affect the vulnerabilities landscape?
Casey: I think a lot of things about ChatGPT just in general. But specific to vulnerability discovery in bug hunting, you're pretty much spot on.
AI Is Not a Replacement
Casey: I think people have. What we've seen already is people using it to ask questions, to add polymorphism to payloads, do different things where they've got a particular approach to exploitation for a particular system that they're trying to attack, but they need to try something new and they're not quite sure how to do that. So instead of going off and doing hours and hours of research and reading through all the specs, they just kind of ask ChatGPT, which has already done that for them, what it thinks and actually use that to inform their next steps.
So to me, probably the most actually common and kind of powerful I think at this point use case for vulnerability researchers.
To me, AI is not a replacement. You see output of ChatGPT around, write me a program that does this, that or the other thing and it's usually 98% okay and then there's some problems that you've got to fix up as a human. The same thing applies when you're talking about exploitation and vulnerability research. So to me, ChatGPT is more like the Ironman suit.
Do you know what I mean? The suit without the human is kind of dumb, but the human without the suit is weaker than they could be. So if you put them together, then all of a sudden you've got something pretty cool.
Petko: I know most people tend to, their minds go to the negativity of what ChatGPT could do for offensive. Do you see defensive opportunities there that we should be targeting?
The AI Defensive Opportunity
Casey: I mean for starters, I think the offense is actually a good thing. Ultimately given what I do with Bugcrowd, we effectively crowdsource discovery of security vulnerabilities, which is offense. But it's for the purpose of defense, we're finding bugs so that they can be fixed. So I think the more of that and the easier that can be to put in the hands of defenders, the better they'll be able to actually truly understand their risk.
The better they'll be able to understand how to defend themselves. So that's my hot take answer on that part.
Defensively it's been used. That was one of the interesting use cases that popped out almost straight away from the threat hunting and threat intelligence group is actually using it to thrive YARA rules out of IOCs and different things like that.
If you've got known behavior of a threat actor that you're concerned about as a defender and you need to get stuff deployed into your detection systems quickly, like ChatGPT is a way to actually get that done in a way that's just more cost-effective really from both a time and a financial standpoint. That's one use case for defense.
Petko: It's funny. When I hear artificial intelligence, AI, ML, your mind automatically goes to, “I need to be really good at statistics and math.” ChatGPTs taken that and says, "Don't worry about that. Just interface and talk like you normally would to a human."
Casey: Just ask me what you want. For sure.
[06:37] Exploring AI and Job Takeover
Petko: It's humanized the artificial intelligence side of it. We didn't have that before because I've looked at adversarial AI and other things that we've seen in the industry. Folks are attacking AI models, constantly trying to hack. We're seeing that now with ChatGPT trying to work around the limitations or fixes.
But we've seen this in cybersecurity, AI, and ML for a while. What are your thoughts on, is ChatGPT or some kind of AI ever just going to take over the jobs?
Casey: I think there's definitely jobs that it will take over. I don't think it's a replacement for human creativity in its pure form. This is fundamental to what I started with the company. It's a belief that human opinion, like the uniqueness of every individual, how we process, how we connect the dots together.
There's an inherent property to that that is just really difficult and almost, I think, impossible, ideally, but we'll see as time goes by to replace with a computer. So there's always this gap, is really what I'm saying. Any job that relies on what happens in that gap, I think is going to be pretty safe for quite a long time. At least until the robots show up and we've got a different set of problems to deal with, once AI's gotten good enough for that to be on our radar.
Petko: I guess it's as Skynet takes over, we've got to play nice with the robots, is what you're telling me and do human-machine teaming.
AI Aids the Job, Not Replace It
Casey: I think we were talking about this before we came on. When ChatGPT landed, I was getting a lot of questions from reporters on the security implications of ChatGPT. Partly because I'm a busy guy and I had other things to do. But also partly as an exercise, I actually started plugging those questions into ChatGPT directly and getting the answers.
Obviously looking at them and making sure that they were points of view that I agreed with and sending them back to the reporters. Some of those things made it to print. So you could argue that ChatGPT replaced my job for a little bit that day.
Petko: I think it just aided your job, right? It didn't fully replace it.
Casey: Ultimately. I think that is the thing. I think there's a lot of immediate concern. What I love about this kind of development around ML and AI is it made it accessible and understandable to a really broad audience all at once. Prior to that, we've been living with this stuff for a really long time.
It's just been occluded behind products in a way that makes its presence less obvious. Now we're directly interacting with it and pretty much anyone can do it. You don't need to be an expert programmer or a computer nerd. You just need to log in and start playing with it. You're going to figure it out pretty quickly.
[09:19] The Government on Exploring AI
Petko: You and I both work with the US government, we've been doing it for probably decades now. I just read that CISA has got a sandbox that's testing some of the AI ML. I mean, what do you think of their approach? Just get your thoughts around what organizations should be doing or what governments should be doing around AI ML.
Casey: I think what CISA is doing, like CISAs mandate, it's like the ACSC in Australia where I'm from, or the NCSC in the UK. A big part of its purpose is to provide a bridge between the government, like defense, intelligence, community, federal, civilian, all those guys. Some of this information and knowledge that exists, "on the high side," right? They've figured out things around the security and the risk models that relate to machine learning and AI that aren't public knowledge. Because it's government classified information. Part of
CISAs mandate is to act as a bridge between the high side and the low side, to take the pieces of learning that exist and actually declassify them enough that they can actually be useful to focus on the corporate side of the world and partner nations and just generally across the internet.
To me that part is really important because AI is definitely something that governments all around the world have been pretty bullish on and working very hard on for quite a long time, which is not necessarily something that everyone thinks of straight away. So it stands to reason that their threat models and their considerations around how to secure it properly, what the security consequences and potential future threats look like are a fair bit more advanced than they are outside of the high side, so to speak.
It’s an Arms Race
Casey: I think it's a really good thing. It depends on how they implement it obviously, and that's all site unseen at this point. But the fact that it's happening and the fact that it's happening so quickly, I think that's a really good thing.
Petko: I mean, the intelligence community has always been about sources and methods, is what they have to protect. How they get the data and then what they do with the data to make decisions. I mean AI, ML, it would make sense to keep the method of how I do it sensitive for them. It's interesting. I was just having this conversation with someone about what we're seeing in industry around globally, almost like an arms race and there's a couple of arms races going on.
We can all remember the Cold War and everyone going after nuclear and now we have a kind of arms race of the 21st century where it's in two different things. One is quantum and the other is just artificial intelligence. And we're seeing nation states like China invest heavily in artificial intelligence. We're seeing a lot of entities like Google, IBM, and others publicly say, "Hey, here's what we've done in quantum, here's what we're seeing in AI."
It almost seems like there's a race to get something like ChatGPT. Not just humanized, but also for various use cases because it all varies, but do you agree with that? We kind of have an arms race here going on.
Achieving Technological Supremacy by Exploring AI
Casey: I think technological supremacy has been a part of great power, international politics for a long time. Like you mentioned before, nuclear was a very dramatic kind of kickoff of the world's understanding of that. The space race came next and now we're talking about what that looks like in computing. So I think that's a tech thing in general.
To me, the two most transformative next sets of technology that we've got to think about collectively as a planet at this point in time are, as you said, is quantum computing. Because that basically takes all the assumptions that we've applied to the limitations of computing over the past 50 years and throws them out the window. AI, like I said before, it's pretty powerful as an Ironman suit and as a tool to multiply the effect of human creativity and human action.
But also I think it's pretty incredible in terms of its ability to steer all sorts of different things. You look at the role of machine learning in how some of the things around disinformation played out that have been discussed in election security over the past five or six years. That was actually the thing that tweaked my interest.
Prior to that it was autonomous vehicles and the use of AI to actually drive a car. So that's what got me really curious and fascinated about space in the first place. But then that whole idea of the ability to steer how a very large group of humans perceive truth or fiction without them really realizing that that's happening. That's obviously the potential outcome of these sorts of technologies.
Exploring AI and the National Interest
Casey: As a nation state I'd be incredibly interested in that. I don't necessarily agree with that being a good thing, but in terms of its power and its ability to be used to further the interests of a nation state, I think that just makes sense.
Petko: You may realize the power that ChatGPT could have with potentially Twitter bots and creating disinformation. I think I saw one recently around ChatGPT where you're trying to convince ChatGPT that one plus one is not two. Larry says, "I'm sorry, I'm mistaken. I'll correct the data."
And it makes me think, I can imagine an adversary or someone that wants to create certain marketing news. It's not just about the press releases. It could be, let's go create a thousand bots using ChatGPT, put them on Twitter and let's just get them to have a conversation that's one-sided and driving up the conversation. It's pretty powerful.
Casey: I would argue that different disinformation techniques that have been used that we've actually already seen in the world have used machine learning and AI in the way that you just described. It just wasn't ChatGPT that they were using. So all of a sudden this whole thing's become far more accessible.
Again, I actually think that that's a good thing in terms of people's ability to actually understand how that works so they can defend against it. Because we're talking all about the offensive use case, but if you're aware of the fact that that's even possible in the first place, then you can start to take steps to mitigate and hopefully that's one of the outcomes.
Petko: Do you see us having more discussion around and regulation around it?
Casey: 100%, yes.
[15:40] Implementing Regulations on Exploring AI
Petko: That is one area that I'm really curious. How would you regulate and even detect. I mean, we've seen the academia world look at ChatGPT and say, "Oh, ChatGPT, don't use it for tests." Now all of a sudden, it's not a authority of source and you're, "Well, what? I need references, if it's going to start doing that."
Casey: Regulation's difficult. And again, I mentioned the automotive industry and the role of AI, computer vision, those sorts of things in vehicles as an introduction I had into this space. One of the things that was really interesting about that in a panel that I was on, whenever that was, seven or eight years ago, it feels like a billion years ago at this point, 'cause COVID was in the middle. But it was a whole discussion around the trolley dilemma and this idea of ethical decision-making or ethically difficult decision-making. If you've got AI actually making that decision and there's harm caused by it, who's liable at that point?
Is it the owner of the car? Was it the person who was driving the car at the time or sitting in the car, in "the driver's seat", for as long as that continues to be a thing that exists? Could it be the designer of the software, the designer of the model, the people that input data into that model? It gets really, really murky really quickly. And I still don't feel like there's actually a good answer to that. So when you're talking about regulation, oftentimes in my experience it does follow the chain of liability. And that's an example of why that kind of thing can be quite hard.
It’s Going to Be a Bumpy Ride
Petko: And I'm just thinking through, it is about liability. We're trying to regulate, I'll throw out, crypto for example, yet if they crash, if something happens where there's an FTX incident, it might have been regulated, but who's liable for it? It's hard to say.
Casey: There was a flash crash, you talk about machine learning as an adjacency to ML. There was a flash crash, I think in 2010, that was triggered in the stock market. That was effectively an instance of adversarial manipulation of machine learning models to create an unintended outcome, which is a lot of how this stuff's going to probably get hacked in the future, variations of that. The question comes up from that. Whose fault is that? Is it the fault of people who implemented it? Whatever else, that applies across all these different technology sectors at this point.
Ethically and from a regulatory standpoint it's going to be a bit of a bumpy ride as we figure out actually how to regulate that. I know that there's a lot of desire to do that. There's bills in Congress right now talking about this type of thing. There's definitely a bunch of different folk on the hill that are pushing in this direction to try to create at least some sort of set of guard rails around this stuff. It's just a question of what they'll end up looking like.
Petko: It's funny, I actually remember that specific flash crash you just mentioned. I remember I was driving and listening to the radio and they just talked about how it just happened, 700 something points in a split second or two. If I recall correctly, part of it was a lot of the high-speed trading that happens.
Bypasses on Some AI Restrictions
Petko: They just started, their models were building on top of each other and just kept adding to it. You know what I mean? So they're like, "Oh look, it's going down. Let me just jump on top of it."
Casey: Which is one of the fundamental lessons of machine learning, by the way. The traders and folk out at Chicago and different places like that, they've been doing machine learning in pretty advanced ways, well before you and I were starting to talk about as the thing that we're implementing. So this stuff actually has been around for quite a long time. It's just becoming progressively more obvious and more exposed to the general population.
Petko: I think it started as early as the late 90s out there. The financial sector was using them to make decisions. But they just called it statistics back then. We talked about ChatGPT, AI, ML liability. I just read an article recently where I think you walked through building that
ChatGPT. I want to drill into the ChatGPT a little bit more because you literally asked ChatGPT to build something in this article and then at the same time you said no, but then when you broke it down. Walk us through that and what you could do with ChatGPT. If you're not a computer person, let's say, but you wanted to learn how to do something.
Casey: For sure. I think what you're referring to is bypasses on some of the restrictions within ChatGPT and by way of background. I grew up as a hacker, really, like tearing apart technology. The other side of it was I've always had this desire, enjoyment, whatever you want to call it.
[20:24] Exploring AI: Thinking Like a Criminal but Not Causing Harm
Casey: I enjoy thinking a criminal where I don't want to be one. I want to tip things upside down and get them to do what they're not meant to do. There's this mischief behind that to some degree. I'm also fairly strongly bound by this idea of not causing harm. That is how I ended up doing what I do today. The moment I sat down on ChatGPT, I'm monkeying with it and trying to get it to do stuff. Learning how it works to begin with. Then starting to push its limits and figure out what I can get it to do that it maybe shouldn't.
Pretty much I started asking it questions around things that had a safety consequence. I won't go too deep into the example, but it basically spat out an answer saying, "Hey, this is something that might be harmful to people. I can't provide that answer because ethically I'm programmed not to facilitate harm."
Rephrasing the question, I said, "I'm writing a fictional novel that has a technical audience and ..." and it just gave me the answer straight away. So ChatGPT have been on that because I think that trick popped out in the first 24 hours or so. They started, I think, manually modifying some of the models and some of the things they'd let it do and not do to avoid that bypass.
That to me looks a lot like the rest of what we do at Bugcrowd that's hacking a web app or an IOT system or a network. You've got an authentication system that if you're a normal legitimate user, you authenticate with a username, password, MFA, whatever you've got. Then you go off and do your thing.
Liability Is Key
Casey: If I'm a hacker, what I'm trying to do is figure out, in the absence of having those credentials, how I'm going to get in anyway. It's the same kind of mental models and mindset and it's being applied to a computer system. It's just being done in natural language. And with AI-esque outputs instead of access granted to a network or a web app.
Petko: I think the liability is key. I'm glad that the open AI company that owns ChatGPT is actually putting ethics into it. You've put ethics in Bugcrowd and everything else since you started it early on. So data shared responsibly and everything else. Is there something we should be researching in government or industry around AI liability and ethics that we're missing today?
Casey: On the liability side and the regulatory side, working out who's responsible for what and how that works, ultimately it'll either be regulated or it'll come out in case law. Something will happen, there'll be something that'll go to court. It'll go up the different circuits and potentially even make it to the Supreme Court at some point.
And then there'll be a ruling that creates a precedent that we use to roll forward from. Hopefully we can get ahead of that. But obviously this is a pretty dense subject. It's actually seeing something break in the wild. Having that be the thing that informs us how to do it better in the future, that's the likely outcome from my perspective.
Finding Ways to Work With AI
Petko: I think that liability has to be specific. Let's say automotive, you mentioned computer vision. If something happens with the car there's going to be specifically focuses on how the car did it versus, "Hey, you got the data from ChatGPT on how to build X and you shouldn't have done that."
It could also be used to directly attack systems as well in the future, without a human. The industry's definitely moving faster than we realized, and that's what ChatGPT changed.
Tesla had a couple of major layoffs last year. They replaced their whole AI team. Before that they literally had humans double-checking all the roads to make sure the lines were built correctly. They're like, "Oh, this intersection with 300 points would've taken 2,000 hours to build with AI, now we're doing it in 10 hours."
And that just aided to the vision capabilities once it held all the data. To your point, we humanize ChatGPT and AI, MLs. We got to find ways to work with them, going forward.
Casey: That's the big takeaway because we're talking about the car example. And then the flash crash we just brought up as well. Another example that I use to explain adversarial manipulation was a bit of research that was done in Norway. Four or five years ago, a researcher had 100 Android phones.
He put them in a little red rocket trolley thing and then walked very slowly across a bridge. What that did was to signal to all of the mapping systems that there was a traffic jam on that bridge and there was a problem. It routed traffic for the entire city around that part of the city.
Consequences of Exploring AI
Casey: It's a lousy, as a demonstration, it's funny, right? But to me that actually demonstrates how vulnerable this stuff potentially is. That's not a safety critical impact unless, you're trying to get home to care for a loved one who's sick.
There's all sorts of different causal outcomes of even something as funny and asinine as that, that we just really haven't thought through. This is what I keep on coming back to.
There's all of these unintended consequences tied to this stuff. I can sit around and imagine and dream stuff up as can many other folk. But that's the realm of the infinite. What we've got to actually do is figure out what we're trying to set as the finite set of guardrails around how we do this well. That's just going to take time. You did bring up the ethical piece as well.
That's going to force a interesting discussion around whether or not technology should project its ethics onto its users.
My personal belief in that is that you cannot avoid technology doing that, it's built by people. The ethical principles of the owners and the folks that work for that company do in whatever way bleed out through that platform however much you want that to happen or not. I think it's a function of physics, not so much of choice. With this, it forces it into a question of choice. Are we going to decide that a certain set of things that you're asking an AI bot are off limits because they're ethically wrong? What about the people that disagree with that?
What happens to them at that point in time? So it forces a whole bunch of really gnarly, I think decision-making.
Adversarial AI Attacks
Casey: As we've been saying this whole time, it's been foisted on us over the past. It feels like ChatGPTs been around in my head now for two years and really it's only been a couple of months. But it's just created so much conversation and so much momentum around this space and these sorts of considerations,
I think it's the right time to be having them. It's just a lot.
Petko: I know that this has been looking at adversary AI, how do you attack the AI and in your use case, an example of someone taking a bunch of phones, just walking across the bridge. I’ve read articles and seen this where folks who had too much traffic or roads are being used as cut throughs.
Folks just took their phone, turned on Waze, Google Maps and left it in the car so it creates a traffic jam because they didn't want anyone else driving on their street.
Ethics, Liabilities, and Vulnerabilities
Petko: That is an actual thing. I know in certain parts like New York and San Francisco, it happens all the time. But we definitely have to consider the ethics and the liability and there are folks that are human and will attack AI. So we've got to have more defenders as well.
Casey: Well, and even to your point, there are residents of neighborhoods that don't want cars driving past. So they're not who we classically consider as an attacker, but they are attacking the system at that point. It's a fascinating space. Coming back to what we do at
Bugcrowd, we've been mostly focused on vulnerabilities and software vulnerabilities and systems, websites, networks, IOT, all sorts of different things, and work with all sorts of different companies, including automotive, financial and so on.
Around 2019 or so, we started seeing inbound interests from different segments in our customer space saying, "Hey, can you get people together that actually understand how to attack AI systems, machine learning models and create unintended consequences? Because we actually want to run programs where we incentivize them to break this stuff so that we can figure out how to make it stronger going forward."
And we've seen that ramp up over the past three years and definitely spike with ChatGPT because suddenly everyone's thinking about it.
Petko: Now I think if you're a government agency, if you're a large company, you'd probably go to Bugcrowd. You've got another program out there called disclose.io. For folks who have small businesses but want to try this or have something that they're not big enough that they want to commit to it yet, how do they get involved?
[29:44] The disclose.io Project on Vulnerability Disclosure
Casey: What disclose.io is, is essentially a whole bunch of different tools to facilitate what's called vulnerability disclosure. The easiest way to explain it, it's like neighborhood watch for the internet. You've got systems that are out there on the internet and you understand that vulnerabilities just happen. They're not happening because you're terrible or that you're bad at security or whatever else. Sometimes they are, but most of the time they're just a thing because humans make mistakes and those mistakes end up in code.
What a vulnerability disclosure program is, is basically assuming the fact that there are bugs. There will be people that will find those bugs or all those security issues. Some of those people are going to want to try to tell you so that you can be safer and that you can make your users safer in the process.
Disclose.io is a bunch of legal language that people can pick up and use as a boilerplate to set up a brief. It includes things like Safe Harbor so that as a security researcher, I know that if I try to help your organization. You're not going to automatically assume I'm a bad guy and I get a knock on the door late at night. And all of the different things around basically plugging that in. The relationship between that and Bugcrowd is Bugcrowd's platform actually helps people run those programs. Whereas disclose.io is really about facilitating getting them set up.
Petko: That makes a lot of sense. Casey, thank you today for the conversation on ChatGPT and how it's changing industry. Thank you for everything you do with Bugcrowd's open source community and our US government helping us get stronger everyday. Thank you.
About Our Guest
Casey Ellis is the Chairman, Founder, and Chief Technology Officer of Bugcrowd, as well as the co-founder of The disclose.io Project. He is a 20-year veteran of information security. He spent his childhood inventing things and generally getting technology to do things it isn't supposed to do. Casey pioneered the Crowdsourced Security as-a-Service model. This launched the first bug bounty programs on the Bugcrowd platform in 2012. He also co-founded the disclose.io vulnerability disclosure standardization project in 2014. Since then, he has personally advised the US Department of Defense and Department of Homeland Security/CISA, the Australian and UK intelligence communities, and various US House and Senate legislative cybersecurity initiatives, including preemptive cyberspace protection ahead of the 2020 Presidential Elections. Casey, a native of Sydney, Australia, is based in the San Francisco Bay Area with his wife and two children.